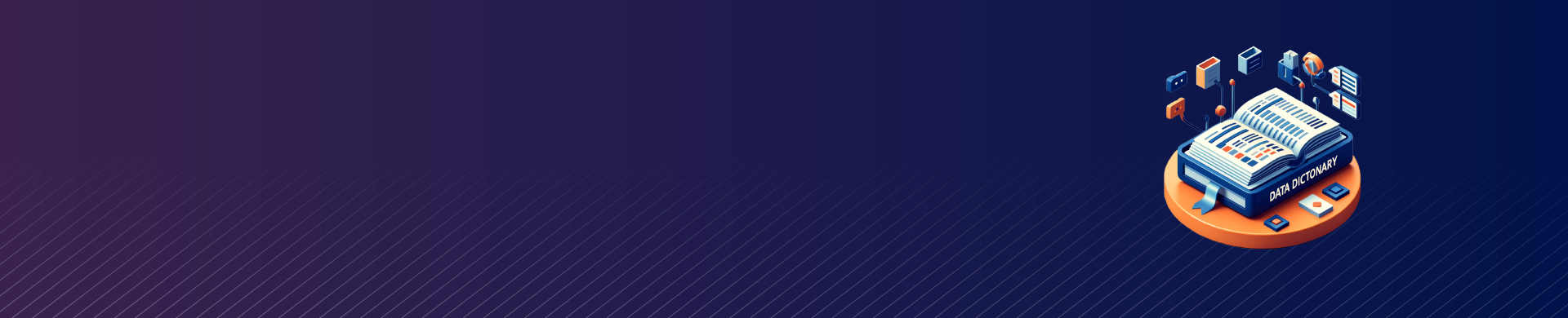
Data Dictionary
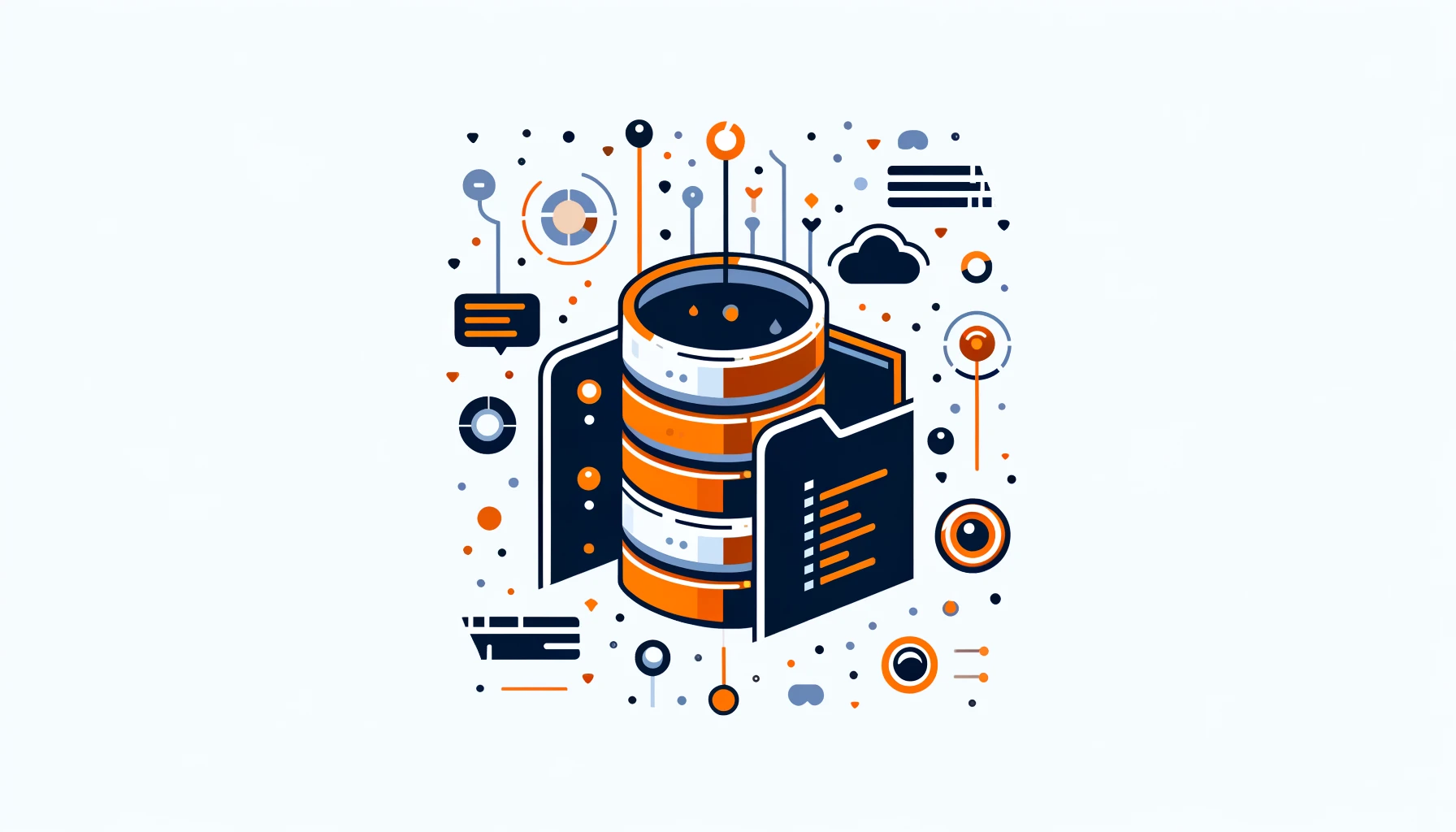
In today’s data-driven world, organizations are collecting and storing vast amounts of information every day. However, without proper management and organization, this data can quickly become a liability rather than an asset. This is where data dictionary comes in.
Using powerful tools for data management is important. These tools help maintain consistent, clear and efficient data. This, in turn, helps organizations make the most of their data assets.
At its core, a data dictionary is a centralized repository of information about an organization’s data. It contains metadata about the definition, naming, and attributes of data elements within a database or data pipeline. Data dictionaries help prevent mistakes and disagreements by giving one reliable place for all data information. This stops confusion and errors that can happen when people have different ways of discussing data.
The Importance of Data Dictionaries in Data Engineering
Data engineering is the backbone of any data-driven organization. It includes creating, building, and managing data pipelines and databases for organizations to gather, store, and analyze their data. However, without clear and consistent definitions of data elements, data engineering can quickly become a nightmare.
This is where data dictionaries come in. They help define the scope and rules for each data element in a project. They also provide a clear understanding of the data assets involved. This ensures that everyone involved in the project aligns in their understanding and interpretation of the data.
For example, consider a large e-commerce company that collects data on customer purchases, website interactions, and shipping information. Without a data dictionary, different teams may use different names or meanings for the same data within the organization. The marketing team may refer to a customer’s total purchase amount as “revenue,” while the finance team calls it “sales”. This lack of consistency can lead to confusion, errors, and missed opportunities for analyzing.
Data Dictionary Class Implementation Example
class DataDictionary: def __init__(self): self.elements = {} def add_element(self, name, data_type, description, format=None, constraints=None): self.elements[name] = { 'data_type': data_type, 'description': description, 'format': format, 'constraints': constraints } def get_element(self, name): return self.elements.get(name, None) def update_element(self, name, **kwargs): if name in self.elements: self.elements[name].update(kwargs) def remove_element(self, name): self.elements.pop(name, None) # Usage example dd = DataDictionary() # Adding elements dd.add_element('customer_id', 'integer', 'Unique identifier for a customer', constraints='PRIMARY KEY') dd.add_element('first_name', 'string', 'Customer\'s first name', format='VARCHAR(50)') dd.add_element('last_name', 'string', 'Customer\'s last name', format='VARCHAR(50)') dd.add_element('email', 'string', 'Customer\'s email address', format='VARCHAR(100)', constraints='UNIQUE') # Retrieving an element print(dd.get_element('customer_id')) # Updating an element dd.update_element('email', description='Customer\'s primary email address') # Removing an element dd.remove_element('last_name')
A data dictionary helps employees at e-commerce companies. It provides consistent terms and definitions for each data element and its attributes. This means that everyone in the company will understand and interpret the data in the same way. It ensures that there is no confusion or miscommunication when discussing data.
Here’s a table that illustrates the content of a data dictionary:
Data Asset Name | Data Type | Format | Description |
---|---|---|---|
customer_id | Integer | INT | Unique identifier for a customer |
first_name | String | VARCHAR(50) | Customer’s first name |
last_name | String | VARCHAR(50) | Customer’s last name |
String | VARCHAR(100) | Customer’s email address | |
purchase_id | Integer | INT | Unique identifier for a purchase |
product_id | Integer | INT | Unique identifier for a product |
Having a clear data dictionary is essential for effective communication and decision-making within the company. This consistency makes it easier to combine data from various sources. It also helps in accurately analyzing the data. Finally, it aids in making decisions based on the data.
Data Dictionary and Data Governance
Data governance is the management of an organization’s data assets. It includes policies, procedures, and standards to make sure data is accurate, consistent, and secure.
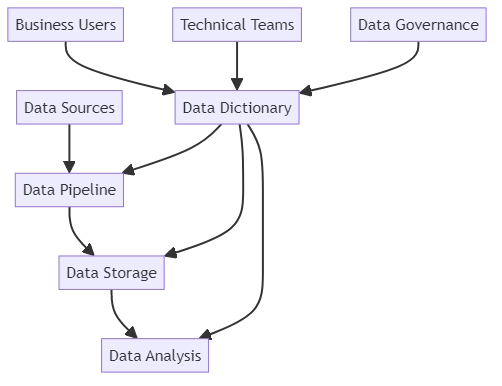
Data dictionaries play a crucial role in data governance. Data catalogs provide a central source of information about an organization’s data assets. This makes it easier to enforce data quality standards, track data lineage, and ensure compliance with regulations and standards.
For example, consider a healthcare organization that is subject to strict data privacy regulations such as HIPAA. The organization can ensure patient information stays safe by listing all data and its importance. This helps ensure only the right people have access to private information.
Data Dictionaries Content
The content may vary depending on the organization and its data assets, but usually includes key elements.
- Data asset name: The unique identifier for each data element, such as customer_id or product_name.
- Formats pertain to the unique method of data storage, like numbers, text, or dates. Ensuring precise data management and analyzing is vital.
- Comprehending data element and resource connections: Investigate each data unit’s links with others in the database or pipeline. For instance, an e-commerce database may connect a purchase_id to a customer_id.
- More information is available in the reference data. This includes the meaning of the element and instructions on how to use it. Provide this additional information to help improve understanding.
- Data quality rules ensure data is accurate and consistent by setting guidelines for valid values and formats.
- Element hierarchy determines the structure and organization of data elements within a larger data asset. For example, it involves understanding the relationship between a main category, such as product_category, and its sub-categories.
- Understanding where you store the data and how can access it. This includes providing the database name or API URL.
By centralizing this information, dictionaries enable stakeholders to quickly find specific data element details without searching multiple sources or consulting different teams.
Active vs. Passive Data Dictionaries
Another important distinction is the contrast between active and passive dictionaries.
Active dictionaries directly link to a specific database and automatically update whenever data changes occur. The dictionary automatically updates to show the most current information. This helps to avoid mistakes and inconsistencies. The database management system itself typically manages active dictionaries, making them a seamless part of the data infrastructure.
For example, consider a financial institution that uses an active data dictionary to manage its customer data. The system automatically updates the dictionary. It includes the name, account number, and contact information of a new customer.
This occurs when we add a new customer. This ensures that everyone within the organization has access to the most up-to-date information about each customer.
Passive dictionaries, on the other hand, do not connect to a specific database. The organization has to update them manually. This takes more work, since users have to update the dictionary by hand whenever the data changes.
But passive data dictionaries are more flexible. Organizations can use them with many different databases. They can also include extra information that the database management system might not record.
For example, a marketing agency may use a passive data dictionary to manage data from multiple clients and campaigns. The dictionary may include information about each client’s branding guidelines, target audience, and messaging strategies, in addition to the standard metadata about data elements. The databases may not store this information. However, it is crucial for ensuring that the agency’s work aligns with each client’s needs and goals.
The Business Value of Data Dictionary
While technical teams primarily use dictionaries, they also provide significant value to business stakeholders. Data dictionaries help connect technical and business aspects of a company by providing a simple overview of its data. This tool assists in understanding the data assets of a company. It helps in bridging the gap between the technical and business aspects of a company.
Business stakeholders can use dictionaries to:
- Capture and store the information they need in the right format and place.
- Find opportunities to make decisions based on data
- Ensure the organization gets the most value from its data assets
For example, consider a retail company that uses dictionaries to manage its inventory and sales data. The company can make sure everyone understands by clearly explaining each piece of information and its features.
This includes the sales team and supply chain managers. This way, everyone will use the same words and meanings. This makes it much easier to track inventory levels, forecast demand, and make informed decisions about pricing and promotions.
Data dictionaries are crucial in outlining specifications for new data pipelines or products. They offer a comprehensive view of the current data environment, enabling stakeholders to spot deficiencies and potential enhancements. This ensures that new projects are in sync with the company’s overarching data strategy.
Healthcare providers can use dictionaries to improve patient care with data-driven insights. Data dictionaries clearly define data elements related to patient health outcomes. This helps providers capture and analyze the right data for clinical decision making and population health management.
Conclusion
Data dictionaries are a critical component of effective data management, providing organizations with a centralized source of information about their data assets. By enforcing consistency, enabling collaboration, and providing valuable insights, dictionaries help organizations get the most value from their data.
Data dictionaries are important tools for organizations that use data to make decisions and grow their business. Organizations can keep their data valuable and strategic in the long term by creating and updating detailed dictionaries.
The importance of effective data management is increasing as data continues to grow in volume, variety, and velocity. Effective data management is becoming more important as data continues to grow rapidly in volume, variety, and velocity. Organizations can set themselves up for success in the data-driven future by using dictionaries. This can help unlock new opportunities for innovation, efficiency, and growth.