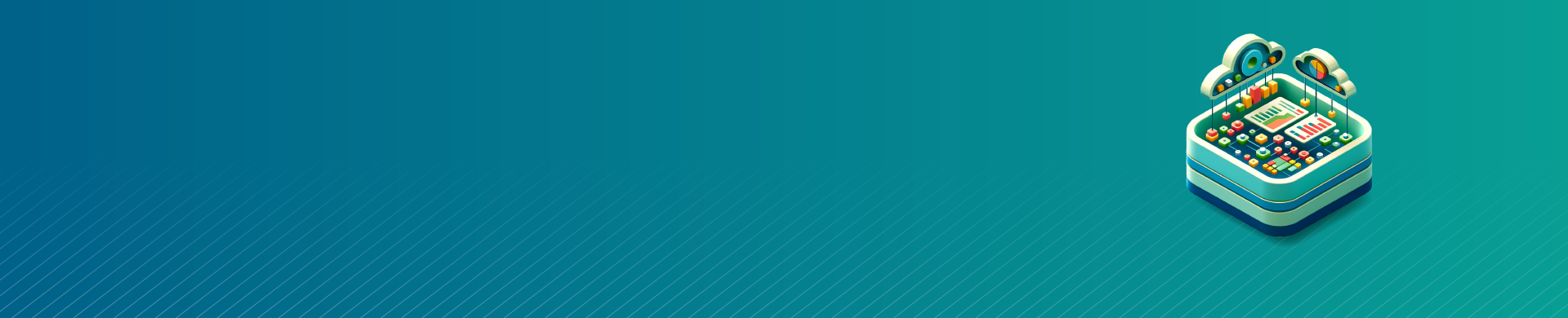
Data Generalization
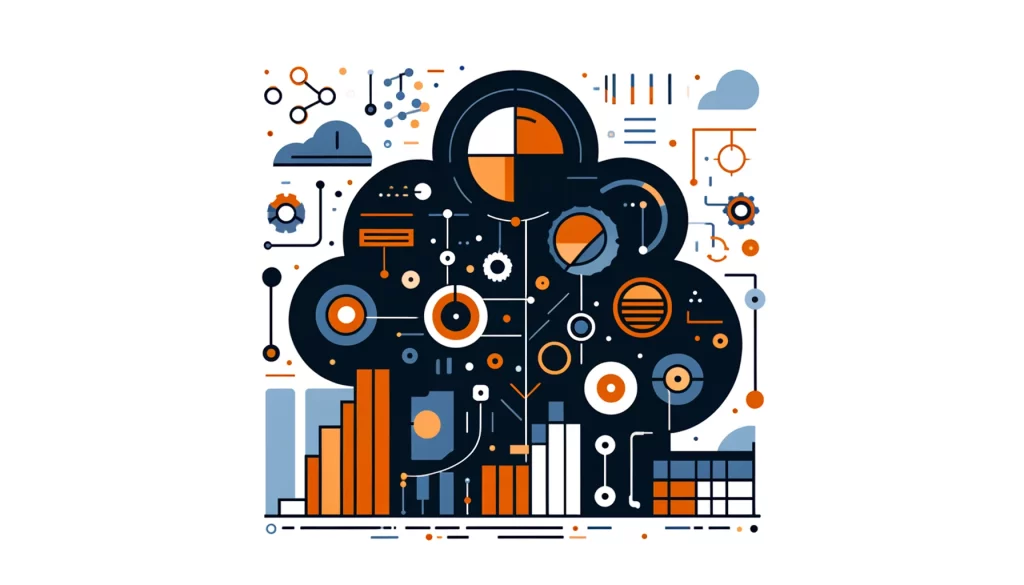
Introduction
In today’s data-driven world, organizations collect and store vast amounts of information. However, handling such large datasets can be challenging, especially when analyzing, storing, and securing them. This is where data generalization comes into play.
In this article, we will discuss generalization, why it’s important, and the security concerns related to it.
What is Data Generalization?
Data generalization is a technique used to create summary information from detailed data. It involves reducing the granularity of data by aggregating or combining individual data points into broader categories or ranges. The goal is to simplify the data while preserving its essential characteristics and patterns.
Let’s consider a dataset containing the ages of individuals. Instead of storing each person’s exact age, we can generalize the data by creating age groups such as “0-10”, “11-20”, “21-30”, and so on. This way, we reduce the level of detail while still maintaining the overall distribution of ages.
Forms of Data Generalization:
- Aggregation: Grouping data points based on common attributes or ranges.
- Binning: Dividing continuous data into discrete intervals or bins.
- Rounding: Approximating numerical values to a specified precision.
- Sampling: Selecting a representative subset of data from a larger dataset.
Importance of Data Generalization:
- Improved Performance: Generalized data consumes less storage space and processes faster, improving overall system performance.
- Easier Analyzing: Summarized data is easier to comprehend and analyze, enabling quicker insights and decision-making.
- Privacy Protection: Generalizing sensitive data helps protect individual privacy by reducing the risk of identification.
- Compliance: Generalization techniques can help organizations comply with protection regulations like GDPR and HIPAA.
Security Aspects of Data Generalization
Data generalization plays a crucial role in ensuring security. By reducing the granularity of sensitive information, it becomes more difficult for unauthorized individuals to identify specific individuals or reveal confidential details. However, it’s essential to strike a balance between data utility and privacy protection.
Consider a healthcare database containing patient records. Instead of saving exact birth dates, the database can save just the birth year or age range to simplify the information. This approach helps protect patient privacy while still allowing for meaningful analyzing.
Implementing Data Generalization
To implement generalization effectively, organizations need robust management tools. DataSunrise offers exceptional and flexible solutions for data security, audit rules, masking, and compliance. Their comprehensive suite of tools empowers businesses to generalize data seamlessly while maintaining data integrity and security.
Conclusion
Data generalization is a powerful technique that simplifies datasets, improves performance, and enhances security. Organizations can make better decisions about data management and protection by understanding generalization basics, importance, and security aspects. Using DataSunrise tools and effective strategies can help businesses utilize data effectively and protect sensitive information.
Request an online demo of DataSunrise’s advanced data management solutions. Discover how DataSunrise can help you generalize data efficiently, ensure compliance, and fortify your data security posture.