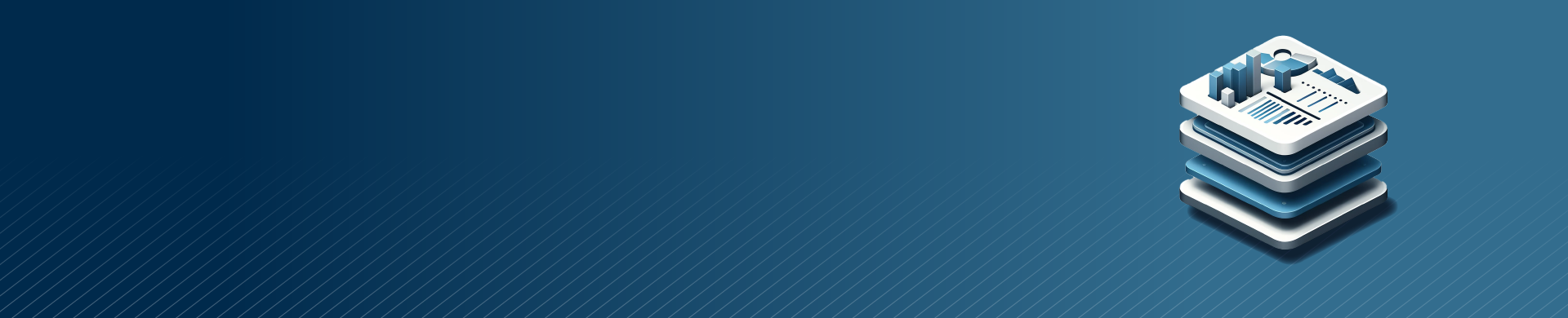
Flattening Data: Simplifying Complex Structures
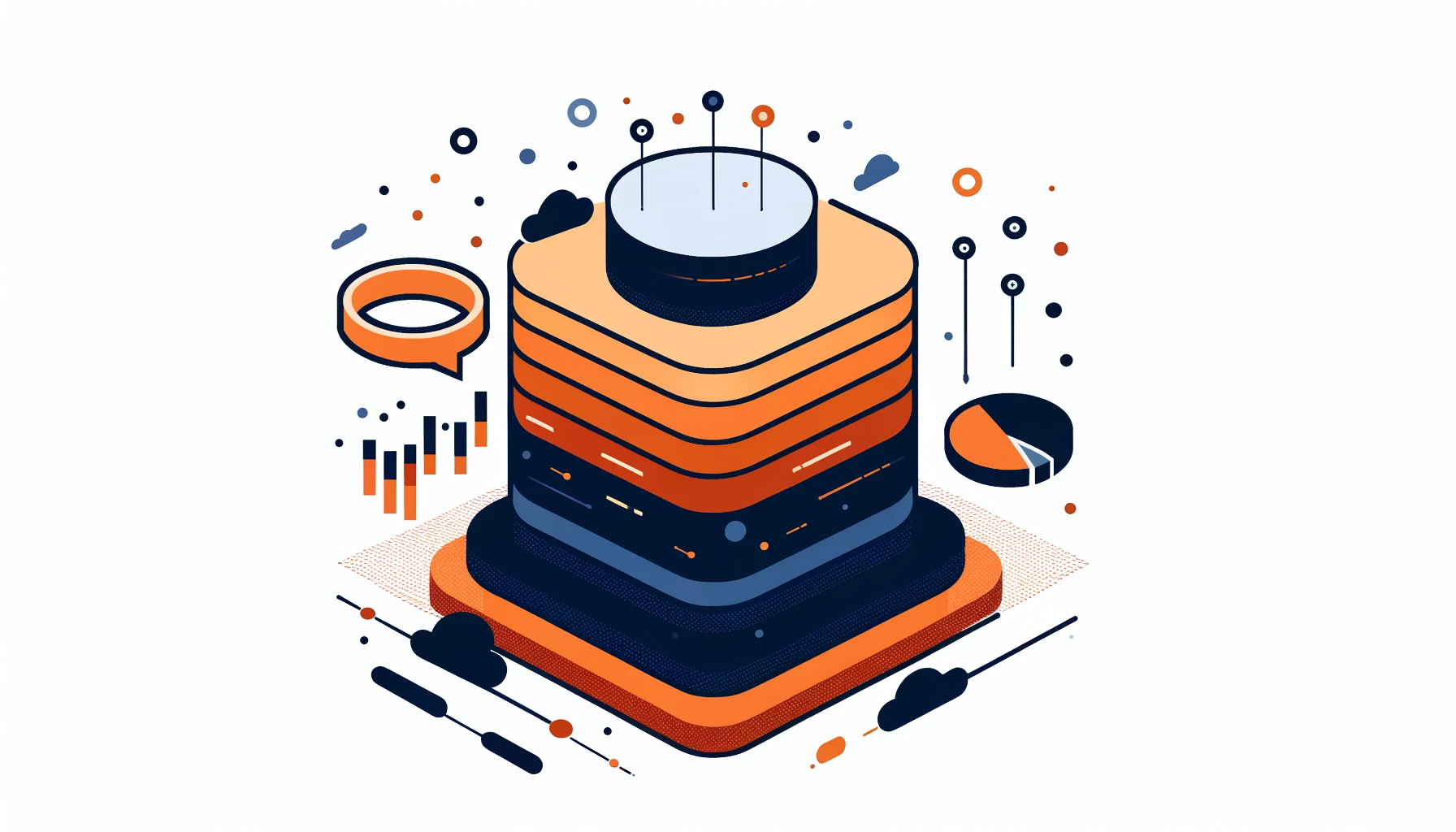
In the world of data processing and analysis, dealing with hierarchical and multidimensional data structures can be challenging. Flattening is a technique that simplifies these complex data structures by converting them into a flat, two-dimensional format.
This article will explain how to flatten data, its main concepts, and workflows for organizing hierarchical and multidimensional data. It will also cover data with relationships. We will also discuss flattening using specific libraries and plain Python, providing examples along the way.
Understanding Flattening
Flattening is the process of converting a hierarchical or multidimensional data structure into a flat, tabular format. It means turning nested or hierarchical relationships into one level, so the data is simpler to handle and study. Flattening is useful when working with JSON, XML, or other structured data that has nested elements or relationships.”
Flattening Hierarchical Data
Hierarchical data consists of parent-child relationships, where each element can have one or more child elements. To flatten hierarchical data, we need to traverse the tree-like structure and extract the relevant information. Here’s an example using Python:
def flatten_hierarchical_data(data): flattened_data = [] def traverse(node, prefix=''): for key, value in node.items(): if isinstance(value, dict): traverse(value, prefix + key + '_') else: flattened_data.append((prefix + key, value)) traverse(data) return flattened_data # Example usage hierarchical_data = { 'person': { 'name': 'John', 'age': 30, 'address': { 'street': '123 Main St', 'city': 'New York' } } } flattened_data = flatten_hierarchical_data(hierarchical_data) print(flattened_data)
Output:
[('person_name', 'John'), ('person_age', 30), ('person_address_street', '123 Main St'), ('person_address_city', 'New York')]
In this example, we define a flatten_hierarchical_data function that takes a hierarchical data structure as input. It uses a recursive traverse function to go through nested elements. The function combines keys with an underscore separator to flatten them. The function returns the resulting flattened data as a list of key-value pairs.
Flattening Multidimensional Data
Multidimensional data consists of multiple dimensions or attributes, often represented as arrays or matrices. Flattening multidimensional data involves converting it into a two-dimensional format. Here’s an example using the numpy library in Python:
import numpy as np multidimensional_data = np.array([ [[1, 2], [3, 4]], [[5, 6], [7, 8]] ]) flattened_data = multidimensional_data.reshape(-1, multidimensional_data.shape[-1]) print(flattened_data)
Output:
[[1 2] [3 4] [5 6] [7 8]]
In this example, we have a 3-dimensional array multidimensional_data. By using the reshape function from numpy, we flatten the array into a 2-dimensional format. The -1 parameter in reshape automatically calculates the number of rows based on the total number of elements and the specified number of columns.
Flattening Data with Relations
In relational databases, a joined SELECT statement combines data from multiple tables using their defined relationships with foreign keys. This allows for querying data from different tables that are linked together.
Databases establish relationships using foreign keys that refer to primary keys in other tables. Using JOINed SELECT statements allows users to retrieve related data from multiple tables in a single query. This creates a denormalized view of the data.
A joined SELECT statement combines data from different tables into one result set. However, it is not exactly the same as flattening. Flattening, in the strictest sense, is different from a joined SELECT statement.
Flattening means changing data structure, like turning nested JSON or XML into a simple table. We will not describe here the situation when a relational database contains nested JSON data as this breaks normalization. But note that MySQL and PostgreSQL RDMS include the JSON tools.
Flattening involves denormalizing data with relationships or foreign key references by combining related information into a single table. Here’s an example using SQL:
The example works with the following data:
-- Create the customers table CREATE TABLE customers ( customer_id INT PRIMARY KEY, name VARCHAR(100) ); -- Create the orders table CREATE TABLE orders ( order_id INT PRIMARY KEY, customer_id INT, order_date DATE, FOREIGN KEY (customer_id) REFERENCES customers(customer_id) ); -- Create the products table CREATE TABLE products ( product_id INT PRIMARY KEY, name VARCHAR(100) ); -- Create the order_items table CREATE TABLE order_items ( order_id INT, product_id INT, quantity INT, PRIMARY KEY (order_id, product_id), FOREIGN KEY (order_id) REFERENCES orders(order_id), FOREIGN KEY (product_id) REFERENCES products(product_id) ); -- Insert sample data into the customers table INSERT INTO customers (customer_id, name) VALUES (1, 'John Doe'), (2, 'Jane Smith'); -- Insert sample data into the orders table INSERT INTO orders (order_id, customer_id, order_date) VALUES (1, 1, '2023-05-01'), (2, 1, '2023-05-02'), (3, 2, '2023-05-03'); -- Insert sample data into the products table INSERT INTO products (product_id, name) VALUES (1, 'Product A'), (2, 'Product B'), (3, 'Product C'); -- Insert sample data into the order_items table INSERT INTO order_items (order_id, product_id, quantity) VALUES (1, 1, 2), (1, 2, 1), (2, 2, 3), (3, 1, 1), (3, 3, 2);
Flattening is performed by joined select:
SELECT orders.order_id, orders.customer_id, customers.name AS customer_name, orders.order_date, order_items.product_id, products.name AS product_name, order_items.quantity FROM orders JOIN customers ON orders.customer_id = customers.customer_id JOIN order_items ON orders.order_id = order_items.order_id JOIN products ON order_items.product_id = products.product_id
In this example, we have three tables: orders, customers, and order_items. By using SQL joins, we combine the related information from these tables into a single flattened result set. The flattened data includes the order details along with the corresponding customer and product information.
Flattening with Libraries
Several libraries in Python provide built-in functionality for flattening data structures. One popular library is pandas, which offers the json_normalize function for flattening JSON data.
Here’s an example:
import pandas as pd json_data = [ { 'name': 'John', 'age': 30, 'address': { 'street': '123 Main St', 'city': 'New York' } }, { 'name': 'Jane', 'age': 25, 'address': { 'street': '456 Elm St', 'city': 'London' } } ] flattened_data = pd.json_normalize(json_data) print(flattened_data)
Output:
name age address.street address.city 0 John 30 123 Main St New York 1 Jane 25 456 Elm St London
The json_normalize function in pandas takes a JSON-like data structure and flattens it into a DataFrame. It automatically handles nested fields by using dot notation to create column names.
Conclusion
Flattening is a powerful technique for simplifying hierarchical and multidimensional data structures. By converting complex data into a flat, tabular format, flattening enables easier data processing, analysis, and visualization. Whether you are working with JSON, XML, arrays, or relational data, flattening provides a way to transform the data into a more manageable format.
Throughout this article, we explored the basics of flattening, its main ideas, and workflows for various data structures. We provided examples using plain Python and specific libraries like ‘numpy’ and ‘pandas’. By understanding techniques described, you can effectively handle and analyze complex data structures in your projects.