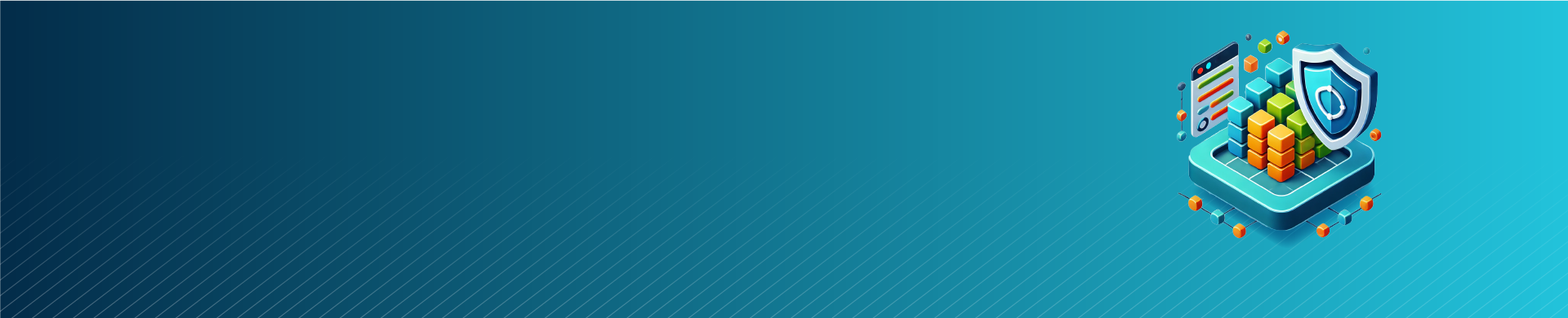
Comprehensive Guide to Data Masking for Dataframe Security and Privacy
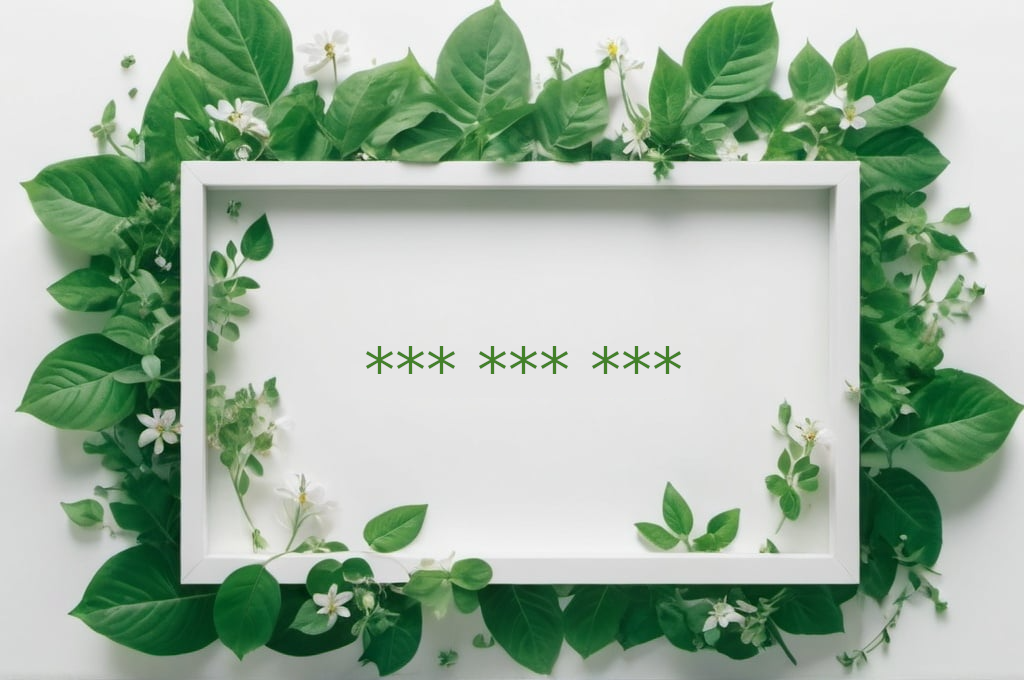
Introduction
You may have come across our articles on data masking from a data storage perspective, where we discussed static, dynamic, and in-place masking techniques. However, the masking procedure in data science differs slightly. While we still need to maintain privacy and provide dataframe data protection, we also aim to derive data-based insights. The challenge lies in keeping the data informative while ensuring its confidentiality.
As organizations rely heavily on data science for insights and decision-making, the need for robust data protection techniques has never been greater. This article delves into the crucial topic of data masking in dataframes, exploring how this procedure safeguards sensitive data while maintaining its utility for analysis.
Understanding Data Masking in Data Science
Data masking is a critical process in the realm of data protection. While we won’t delve too deeply into its general aspects, it’s essential to understand its role in data science.
In the context of data science, masking techniques play a vital role in preserving the statistical characteristics of datasets while concealing sensitive information. This balance is crucial for maintaining data utility while ensuring privacy and compliance with regulatory requirements.
Format Preserved Masking: Balancing Utility and Privacy
Format preserved masking techniques are particularly valuable in data science applications. These methods help maintain the statistical parameters of the dataset while effectively protecting sensitive information. By preserving the format and distribution of the original data, researchers and analysts can work with masked datasets that closely resemble the authentic data, ensuring the validity of their findings without compromising privacy.
What is a Dataframe?
Before diving into masking procedures, let’s clarify what a dataframe is. In data science, a dataframe is a two-dimensional labeled data structure with columns of potentially different types. It’s similar to a spreadsheet or SQL table and is a fundamental tool for data manipulation and analysis in many programming languages, particularly in Python with libraries like Pandas.
Masking Data in Dataframes
When it comes to protecting sensitive information in dataframes, there are two primary approaches:
- Masking during dataframe formation
- Applying masking techniques after dataframe creation
Let’s explore both methods in detail.
Masking During Dataframe Formation
This approach involves applying masking techniques as the data is being loaded into the dataframe. It’s particularly useful when working with large datasets or when you want to ensure that sensitive data never enters your working environment in its raw form.
Example: Masking During CSV Import
Here’s a simple example using Python and pandas to mask sensitive data while importing a CSV file:
import pandas as pd import hashlib def mask_sensitive_data(value): return hashlib.md5(str(value).encode()).hexdigest() # Read CSV file with masking function applied to 'ssn' column df = pd.read_csv('employee_data.csv', converters={'ssn': mask_sensitive_data}) print(df.head())
In this example, we’re using a hash function to mask the ‘ssn’ (Social Security Number) column as the data is being read into the dataframe. The result would be a dataframe where the ‘ssn’ column contains hashed values instead of the original sensitive data.
The output of the code should be as follows:
index name age ssn salary department 0 Tim Hernandez 37 6d528… 144118.53 Marketing 1 Jeff Jones 29 5787e… 73994.32 IT 2 Nathan Watts 64 86975… 45936.64 Sales …
Applying Masking Techniques After Dataframe Creation
This method involves searching for and masking sensitive data within an existing dataframe. It’s useful when you need to work with the original data initially but want to protect it before sharing or storing the results.
Example: Masking Existing Dataframe Columns
Here’s an example of how to mask specific columns in an existing dataframe:
import pandas as pd import numpy as np # Create a sample dataframe df = pd.DataFrame({ 'name': ['Alice', 'Bob', 'Charlie'], 'age': [25, 30, 35], 'ssn': ['123-45-6789', '987-65-4321', '456-78-9012'] }) # Function to mask SSN def mask_ssn(ssn): return 'XXX-XX-' + ssn[-4:] # Apply masking to the 'ssn' column df['ssn'] = df['ssn'].apply(mask_ssn) print(df)
This script creates a sample dataframe and then applies a custom masking function to the ‘ssn’ column. The result is a dataframe where only the last four digits of the SSN are visible, while the rest is masked with ‘X’ characters.
This outputs as follows:
name age ssn 0 Alice 25 XXX-XX-6789 1 Bob 30 XXX-XX-4321 2 Charlie 35 XXX-XX-9012
Advanced Masking Techniques for Dataframes
As we delve deeper into dataframe data protection, it’s important to explore more sophisticated masking techniques that can be applied to various data types and scenarios.
Numeric Data Masking
When dealing with numeric data, preserving statistical properties while masking can be crucial. Here’s an example of how to add noise to numeric data while maintaining its mean and standard deviation:
import pandas as pd import numpy as np # Create a sample dataframe with numeric data df = pd.DataFrame({ 'id': range(1, 1001), 'salary': np.random.normal(50000, 10000, 1000) }) # Function to add noise while preserving mean and std def add_noise(column, noise_level=0.1): noise = np.random.normal(0, column.std() * noise_level, len(column)) return column + noise # Apply noise to the salary column df['masked_salary'] = add_noise(df['salary']) print("Original salary stats:") print(df['salary'].describe()) print("\nMasked salary stats:") print(df['masked_salary'].describe())
This script creates a sample dataframe with salary data, then applies a noise-adding function to mask the salaries. The resulting masked data maintains similar statistical properties to the original, making it useful for analysis while protecting individual values.
Note there are no huge changes in statistical parameters while the sensitive data is preserved as we added the noise to the data.
Original salary stats: count 1000.000000 mean 49844.607421 std 9941.941468 min 18715.835478 25% 43327.385866 50% 49846.432943 75% 56462.098573 max 85107.367406 Name: salary, dtype: float64 Masked salary stats: count 1000.000000 mean 49831.697951 std 10035.846618 min 17616.814547 25% 43129.152589 50% 49558.566315 75% 56587.690976 max 83885.686201 Name: masked_salary, dtype: float64
Normal distributions look like this now:
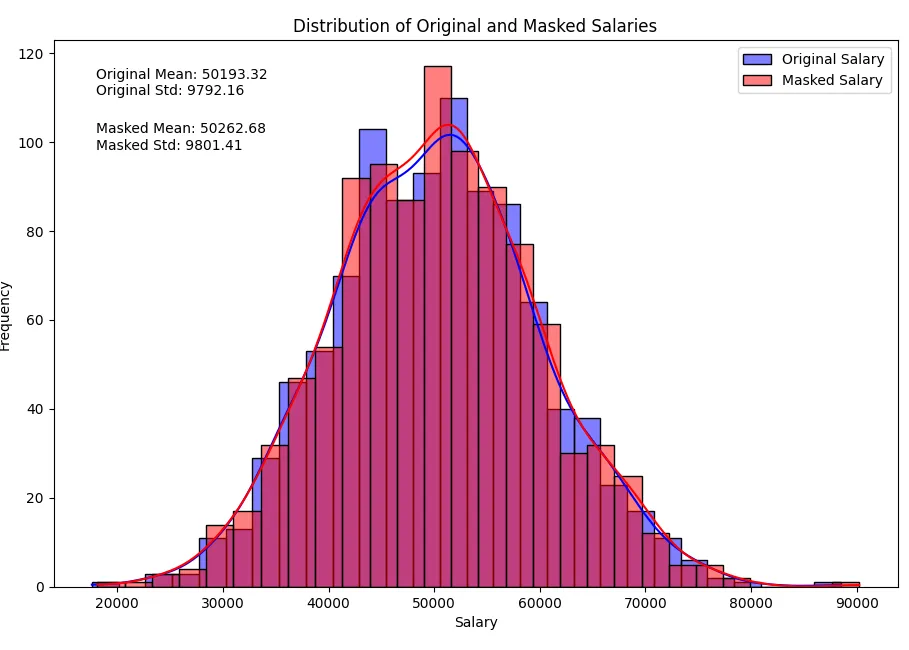
Categorical Data Masking
For categorical data, we might want to preserve the distribution of categories while masking individual values. Here’s an approach using value mapping:
import pandas as pd import numpy as np # Create a sample dataframe with categorical data df = pd.DataFrame({ 'id': range(1, 1001), 'department': np.random.choice(['HR', 'IT', 'Sales', 'Marketing'], 1000) }) # Create a mapping dictionary dept_mapping = { 'HR': 'Dept A', 'IT': 'Dept B', 'Sales': 'Dept C', 'Marketing': 'Dept D' } # Apply mapping to mask department names df['masked_department'] = df['department'].map(dept_mapping) print(df.head()) print("\nOriginal department distribution:") print(df['department'].value_counts(normalize=True)) print("\nMasked department distribution:") print(df['masked_department'].value_counts(normalize=True))
This example demonstrates how to mask categorical data (department names) while maintaining the original distribution of categories.
If you plot the data, it may look as follows. Note that the bar lengths are the same for masked and unmasked data, while the labels are different.
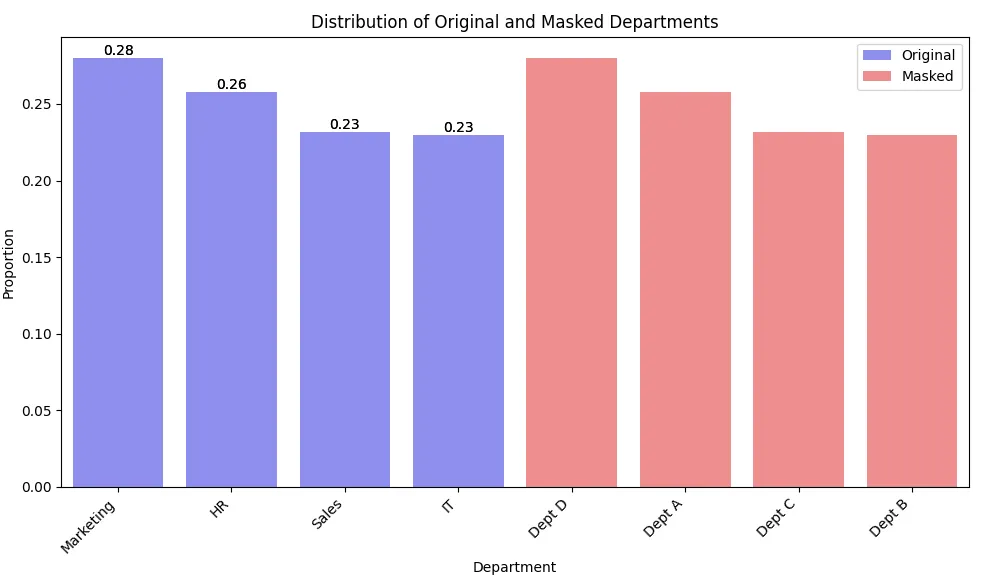
Challenges in Dataframe Data Protection
While masking procedures offer powerful tools for protecting sensitive data in dataframes, they come with their own set of challenges:
- Maintaining Data Utility: Striking the right balance between data protection and usefulness for analysis can be tricky.
- Consistency Across Datasets: Ensuring that masked values are consistent across multiple related dataframes or database tables is crucial for maintaining data integrity.
- Performance Impact: Some masking techniques can be computationally expensive, especially for large datasets.
- Reversibility: In some cases, you may need to unmask the data, which requires careful management of masking keys or algorithms.
Data Masking Best Practices in Data Science
To address these challenges and ensure effective data masking in dataframes, consider the following best practices:
- Understand Your Data: Before applying any masking technique, thoroughly analyze your data to understand its structure, relationships, and sensitivity levels.
- Choose Appropriate Techniques: Select masking methods that are suitable for your specific data types and analysis requirements.
- Preserve Referential Integrity: When masking related datasets, ensure that the masked values maintain the necessary relationships between tables or dataframes.
- Regular Auditing: Periodically review and update your masking procedures to ensure they meet evolving data protection standards and regulations.
- Document Your Process: Maintain clear documentation of your masking procedures for compliance and troubleshooting purposes.
Conclusion
Masking should preserve the data’s property of producing data-driven insights. Data masking in dataframes is a critical aspect of modern data science, balancing the need for insightful analysis with the imperative of data protection. By understanding various masking techniques and applying them judiciously, data scientists can work with sensitive information while maintaining privacy and compliance.
As we’ve explored, there are two approaches to masking data in dataframes, each with its own strengths and considerations. Whether you’re masking data during import or applying techniques to existing dataframes, the key is to choose methods that preserve the utility of your data while effectively protecting sensitive information.
Remember, data protection is an ongoing process. As data science techniques evolve and new privacy challenges emerge, staying informed and adaptable in your approach to dataframe data protection will be crucial.