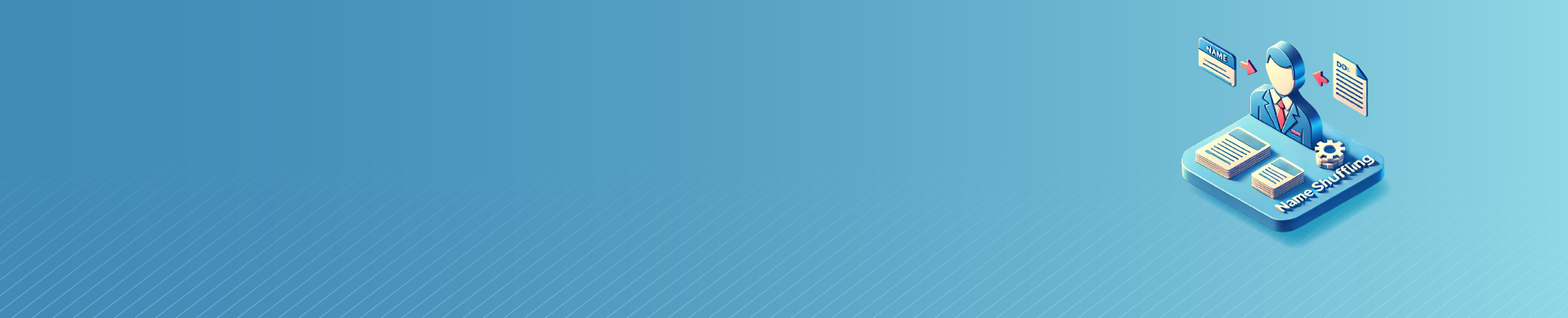
Name Shuffling
Introduction
Companies face the challenge of maintaining data privacy while still using realistic data for testing environments and development. This is where name shuffling and data masking comes into play.
Interesting fact: The SSA (Social Security Administration) releases data on baby names given each year. In a typical year, there are around 30,000 to 35,000 unique names used for newborns.
This article will explore the concept of shuffling, its implementation, and its benefits in creating secure test data.
DataSunrise offers cutting-edge data masking solutions, featuring powerful shuffling techniques. Our advanced platform ensures robust data protection while maintaining data utility. With DataSunrise, organizations can confidently comply with privacy regulations and safeguard sensitive information. Experience the perfect balance of security and usability in your data management processes.
DataSunrise allows for the random selection of values from user-defined lexicons. These lexicons can be created manually or populated with values from the database. This approach implements not only shuffling but also random value selection.
What is Data Masking?
Before diving into name shuffling, let’s briefly touch on data masking. Data masking is a method used to create a structurally similar but inauthentic version of an organization’s data. It replaces sensitive information with realistic but fake data. This allows companies to use masked data for testing, development, and analytics without risking exposure of confidential information.
Understanding Name Shuffling
What is Name Shuffling?
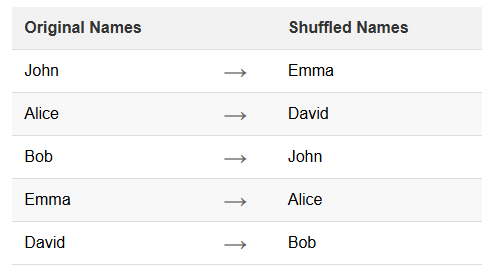
Name shuffling is a specific data masking technique. It involves rearranging existing data within a dataset. This method maintains data integrity and realism while obscuring individual identities. Shuffling is particularly useful for protecting personal information in databases.
As mentioned in Introduction, DataSunrise allows you to create lexicon-based random value selection for masking. The figure below shows the selection of this masking method in the DataSunrise user interface. As you can see, 31,594 values are available, which is much more reliable than simply shuffling a given set. This increased reliability is because when there are n unique values in a column, the probability of any single value being mapped to itself is 1/n.
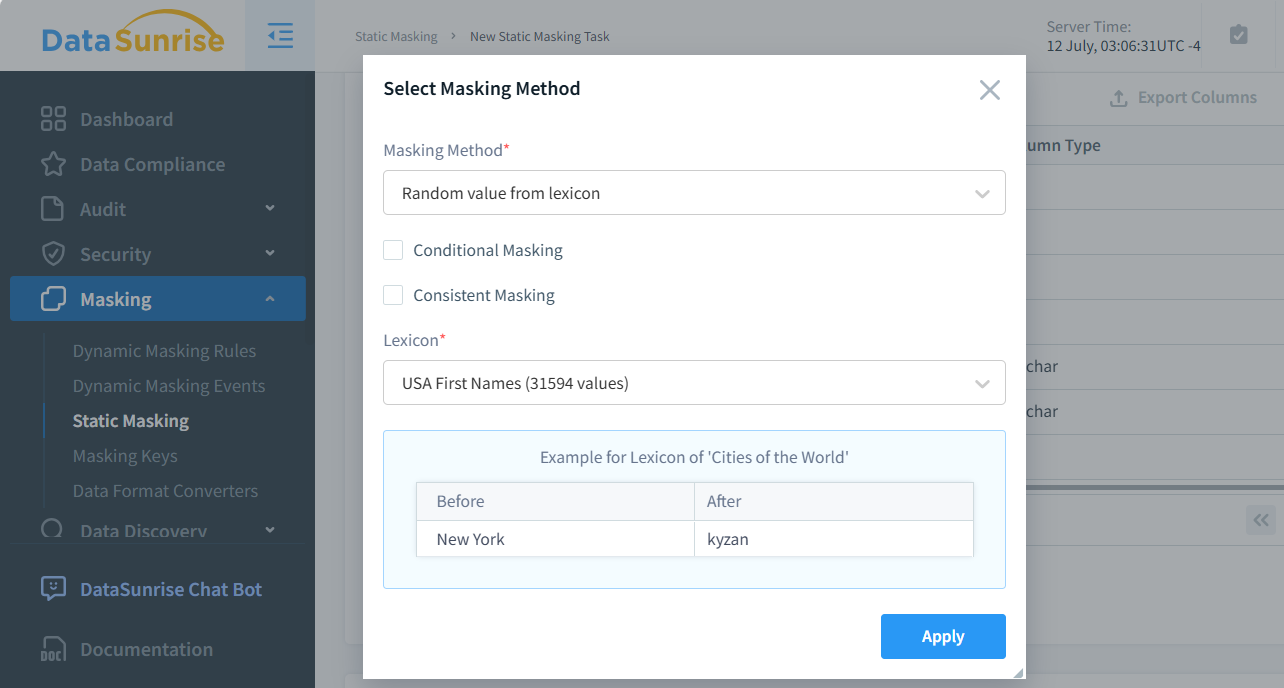
If you prefer to map with existing values, you can easily accomplish this by creating a custom lexicon. This approach is particularly beneficial in situations where the shuffled values are not U.S. first names, as it allows for more contextually appropriate data masking.
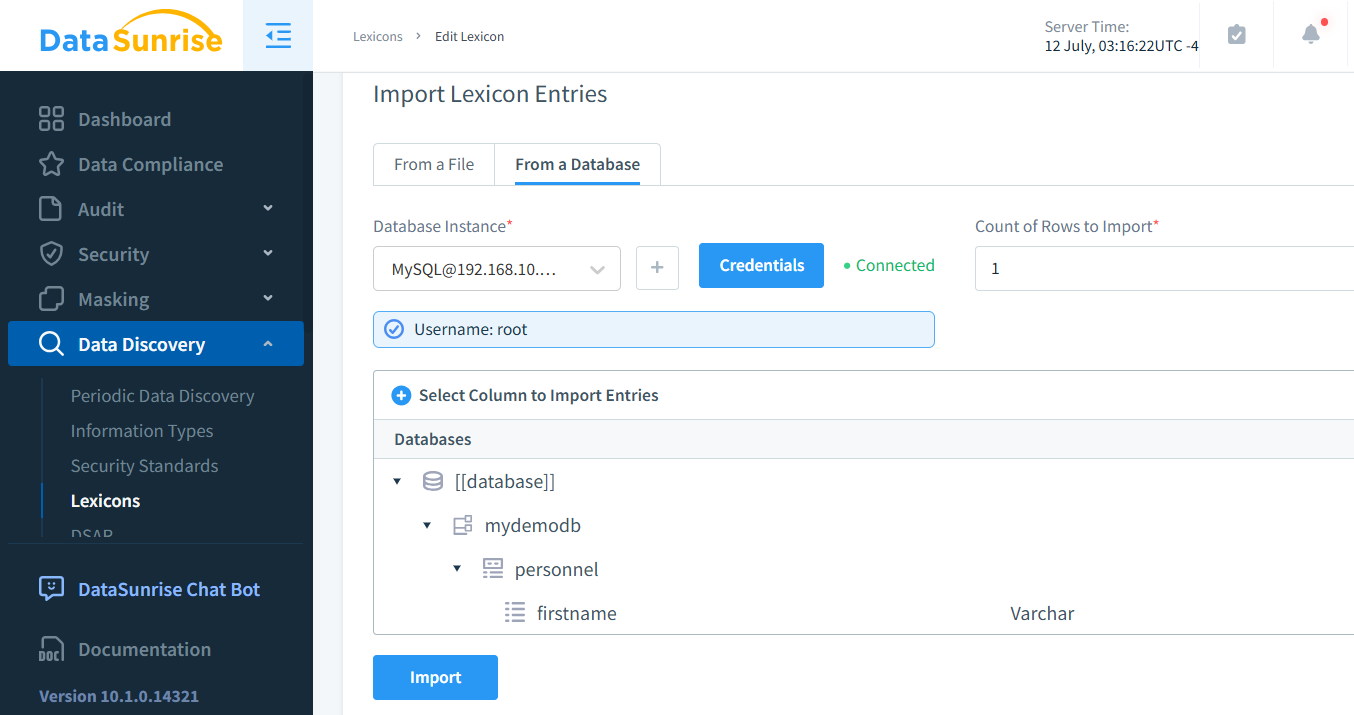
How Does Name Shuffling Work?
The process is straightforward:
- Select a column containing names (first names, last names, or both).
- Randomly reorder the values within that column.
- Replace the original values with the shuffled ones.
This technique preserves the distribution and characteristics of the original data. However, it breaks the connection between individuals and their information.
Implementing Name Shuffling in R and Python
Let’s explore how to implement simplest name shuffling in two popular programming languages: Python and R.
It’s important to note that the level of usability offered by DataSunrise is unparalleled in this context. Creating a flexible, all-in-one solution with just a few lines of code is not feasible using standard programming languages. Our aim here is to highlight the capabilities of specialized tools like DataSunrise compared to general-purpose programming languages.
Name Shuffling in Python
Python offers simple and efficient ways to shuffle data. Here’s an example using pandas, a powerful data manipulation library:
import pandas as pd import numpy as np # Create a sample dataset data = pd.DataFrame({ 'FirstName': ['John', 'Alice', 'Bob', 'Emma', 'David'], 'LastName': ['Smith', 'Johnson', 'Williams', 'Brown', 'Jones'], 'Age': [32, 28, 45, 36, 51], 'Salary': [50000, 60000, 75000, 65000, 80000] }) # Shuffle the FirstName column data['FirstName'] = np.random.permutation(data['FirstName']) # Shuffle the LastName column data['LastName'] = np.random.permutation(data['LastName']) print(data)
This script creates a sample dataset and shuffles both the FirstName and LastName columns. The result maintains the original names but randomizes their order, effectively masking individual identities.
Name Shuffling in R
R also provides straightforward methods for data shuffling. Here’s an example:
# Create a sample dataset data <- data.frame( FirstName = c("John", "Alice", "Bob", "Emma", "David"), LastName = c("Smith", "Johnson", "Williams", "Brown", "Jones"), Age = c(32, 28, 45, 36, 51), Salary = c(50000, 60000, 75000, 65000, 80000) ) # Shuffle the FirstName column data$FirstName <- sample(data$FirstName) # Shuffle the LastName column data$LastName <- sample(data$LastName) print(data)
This R script achieves the same result as the Python example. It shuffles the FirstName and LastName columns, maintaining data integrity while masking individual identities.
Benefits of Name Shuffling
Name shuffling offers several advantages:
- Maintains Data Realism: Shuffled data retains the characteristics of the original dataset.
- Preserves Data Distribution: The frequency of names remains the same, useful for statistical analysis.
- Simple Implementation: It’s easy to apply and understand.
- Reversible: If necessary, the process can be reversed with the right key.
Challenges and Considerations
While name shuffling is effective, it’s important to consider:
- Uniqueness: Rare names might still be identifiable.
- Consistency: Ensure shuffling is consistent across related tables.
- Contextual Information: Other data fields might still reveal identities.
Best Practices for Name Shuffling
To maximize the effectiveness of name shuffling:
- Use Large Datasets: The larger the dataset, the more effective the shuffling.
- Combine Techniques: Use name shuffling with other masking methods for better protection.
- Consistent Application: Apply shuffling consistently across all related data.
- Regular Updates: Periodically re-shuffle data to prevent reverse engineering.
Name Shuffling in Test Data Creation
Name shuffling is particularly valuable in creating test data. It allows developers and testers to work with realistic data without compromising privacy. Here’s why it’s crucial:
- Realistic Testing: Shuffled names maintain the characteristics of real data.
- Privacy Compliance: It helps meet data protection regulations.
- Streamlined Development: Developers can use data that closely mimics production environments.
Conclusion
Name shuffling is a powerful data masking technique. It offers a balance between data utility and privacy protection. By implementing name shuffling, organizations can create realistic test data while safeguarding sensitive information. As worries about data privacy increase, methods like shuffling will become more important in managing data.
For those seeking advanced data masking solutions, DataSunrise offers user-friendly and flexible tools for database security. Our comprehensive dynamic and static data masking tool includes robust shuffling and encryption capabilities. Visit the DataSunrise website for an online demo and explore how our solutions can enhance your data protection strategies.