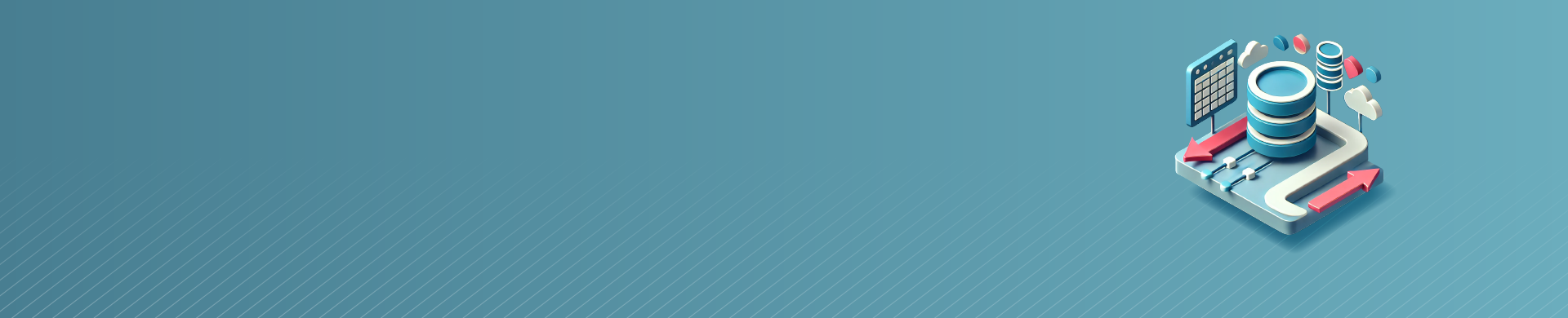
Streamline Data Workflow
For data-driven companies, efficient data processing is crucial for businesses to gain insights and make informed decisions. However, when dealing with sensitive information, it’s essential to balance speed and efficiency with data privacy and security. This article looks at ways to simplify data workflows using ETL and ELT methods while also protecting data privacy.
Understanding Data Processing Streamline Approaches
Before diving into ETL and ELT, let’s examine common data processing streamline approaches:
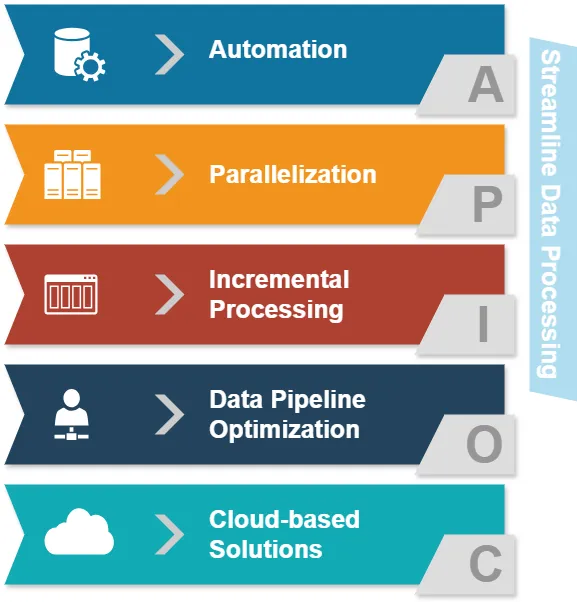
- Automation: Reducing manual interventions in data processing tasks.
- Parallelization: Processing multiple data streams simultaneously.
- Incremental processing: Updating only changed data rather than entire datasets.
- Data pipeline optimization: Ensuring smooth data flow between different stages.
- Cloud-based solutions: Leveraging scalable infrastructure for data processing.
These approaches aim to enhance data processing efficiency. Now, let’s explore how ETL and ELT fit into this landscape.
ETL vs. ELT: A Nutshell Comparison
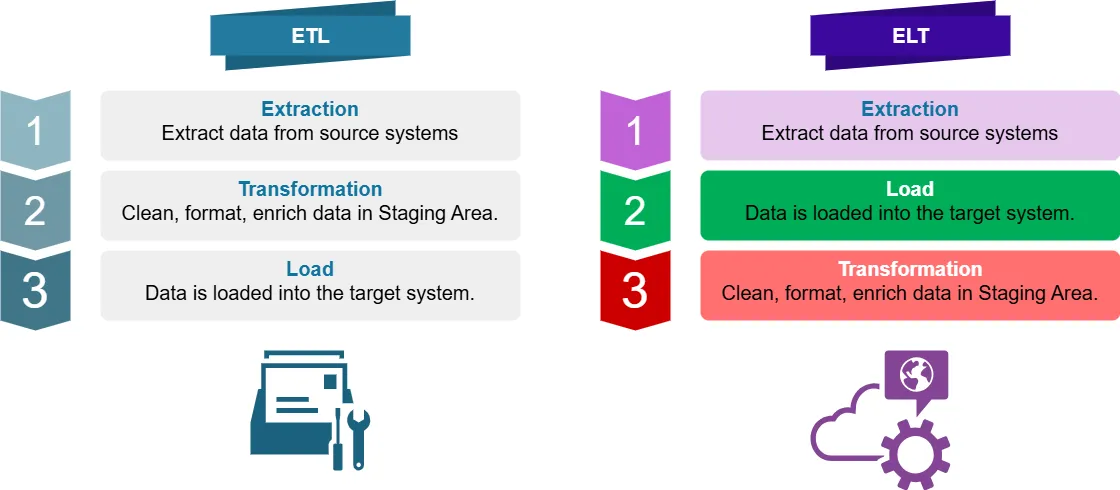
What is ETL?
ETL stands for Extract, Transform, Load. It’s a traditional data integration process where data is:
- Extracted from data source systems
- Transformed (cleaned, formatted, enriched) in a staging area
- Loaded into the target system (e.g., data warehouse)
What is ELT?
ELT stands for Extract, Load, Transform. It’s a modern approach where data is:
- Extracted from source systems
- Loaded directly into the target system
- Transformed within the target system
Key Differences in Optimal Data Processing
For business intelligence, the main difference between ETL and ELT lies in where and when data transformation occurs. This impacts optimal data processing in several ways:
- Processing power: ETL relies on separate transformation servers, while ELT leverages the target system’s power.
- Data flexibility: ELT preserves raw data, allowing for more agile transformations.
- Processing time: ELT can be faster for large datasets due to parallel processing capabilities.
- Data privacy: ETL may offer more control over sensitive data during transformation.
Where Are ETL and ELT Applied?
ETL is commonly used in:
- Traditional data warehousing
- Systems with limited storage or processing power
- Scenarios requiring complex data transformations before loading
ELT is often preferred for:
- Cloud-based data warehouses
- Big data environments
- Real-time or near-real-time data processing
- Situations where raw data preservation is crucial
Streamlining Data Workflows: Python and Pandas Examples
Let’s examine some examples of streamlined and non-streamlined data processing using Python and Pandas.
Non-Streamlined Approach
import pandas as pd # Read data from CSV df = pd.read_csv('large_dataset.csv') # Perform multiple transformations df['new_column'] = df['column_a'] + df['column_b'] df = df[df['category'] == 'important'] df['date'] = pd.to_datetime(df['date']) # Write transformed data to new CSV df.to_csv('transformed_data.csv', index=False)
This approach reads the entire dataset into memory, performs transformations, and then writes the result. For large datasets, this can be memory-intensive and slow.
Streamlined Approach
import pandas as pd # Use chunks to process large datasets chunk_size = 10000 for chunk in pd.read_csv('large_dataset.csv', chunksize=chunk_size): # Perform transformations on each chunk chunk['new_column'] = chunk['column_a'] + chunk['column_b'] chunk = chunk[chunk['category'] == 'important'] chunk['date'] = pd.to_datetime(chunk['date']) # Append transformed chunk to output file chunk.to_csv('transformed_data.csv', mode='a', header=False, index=False)
This streamlined approach processes data in chunks, reducing memory usage and allowing for parallel processing. It’s more efficient for large datasets and can be easily integrated into ETL or ELT workflows.
Data Privacy with ETL and ELT
When dealing with sensitive data, privacy is paramount. Both ETL and ELT can be designed to handle sensitive information securely:
ETL and Data Privacy
- Data masking: Apply masking techniques during the transformation phase.
- Encryption: Encrypt sensitive data before loading it into the target system.
- Access control: Implement strict access controls on the transformation server.
Example of data masking in ETL:
import pandas as pd def mask_sensitive_data(df): df['email'] = df['email'].apply(lambda x: x.split('@')[0][:3] + '***@' + x.split('@')[1]) df['phone'] = df['phone'].apply(lambda x: '***-***-' + x[-4:]) return df # ETL process df = pd.read_csv('source_data.csv') df = mask_sensitive_data(df) # Further transformations... df.to_csv('masked_data.csv', index=False)
ELT and Data Privacy
- Column-level encryption: Encrypt sensitive columns before loading.
- Dynamic data masking: Apply masking rules in the target system.
- Role-based access control: Implement fine-grained access policies in the data warehouse.
Example of column-level encryption in ELT:
import pandas as pd from cryptography.fernet import Fernet def encrypt_column(df, column_name, key): f = Fernet(key) df[column_name] = df[column_name].apply(lambda x: f.encrypt(x.encode()).decode()) return df # Generate encryption key (in practice, securely store and manage this key) key = Fernet.generate_key() # ELT process df = pd.read_csv('source_data.csv') df = encrypt_column(df, 'sensitive_column', key) # Load data into target system df.to_sql('target_table', engine) # Assuming 'engine' is your database connection # Transform data within the target system
Optimizing Data Workflows for Sensitive Data
To streamline data workflows while maintaining data privacy, consider these best practices:
- Data classification: Identify and categorize sensitive data early in the process.
- Minimize data movement: Reduce the number of times sensitive data is transferred between systems.
- Use secure protocols: Employ encryption for data in transit and at rest.
- Implement data governance: Establish clear policies for data handling and access.
- Regular audits: Conduct periodic reviews of your data processing workflows.
Conclusion
It’s important to streamline data workflows. We also need to ensure sensitive information is protected with strong privacy measures. Both ETL and ELT approaches have unique advantages and organizations can optimize them for performance and security.
This article talks about ways organizations can create secure data workflows. These workflows protect sensitive information and allow for valuable insights. Organizations can use strategies and best practices to achieve this goal.
Remember, the choice between ETL and ELT depends on your specific use case, data volume, and privacy requirements. It is important to regularly review and update your data processing strategies. This will ensure that they align with the evolving needs of your business and comply with data protection laws.
For easy-to-use tools that improve database security and compliance in your data processes, check out DataSunrise’s options. Visit our website at DataSunrise to see a demo and learn how we can enhance your data processing. We prioritize keeping your data safe and secure.