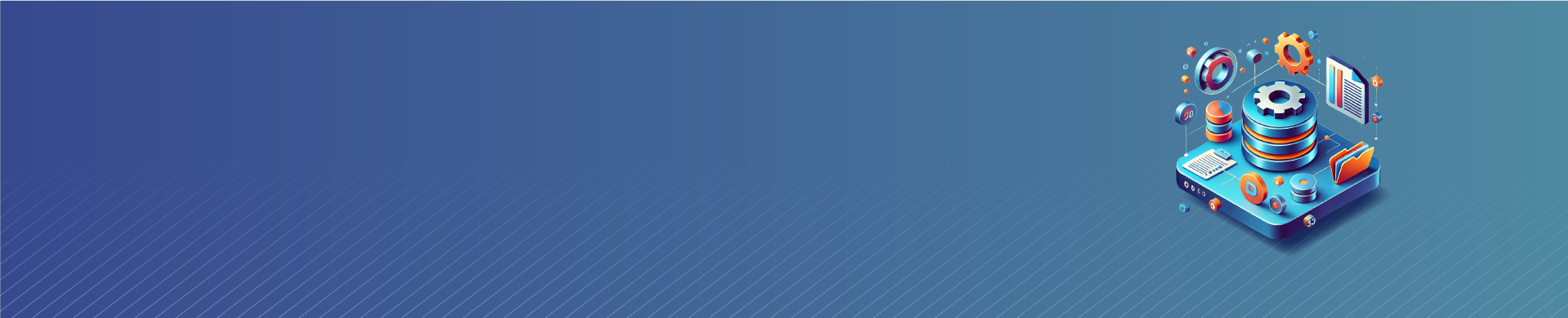
Exploring the Benefits of Synthetic Data Generation for Modern Workflows
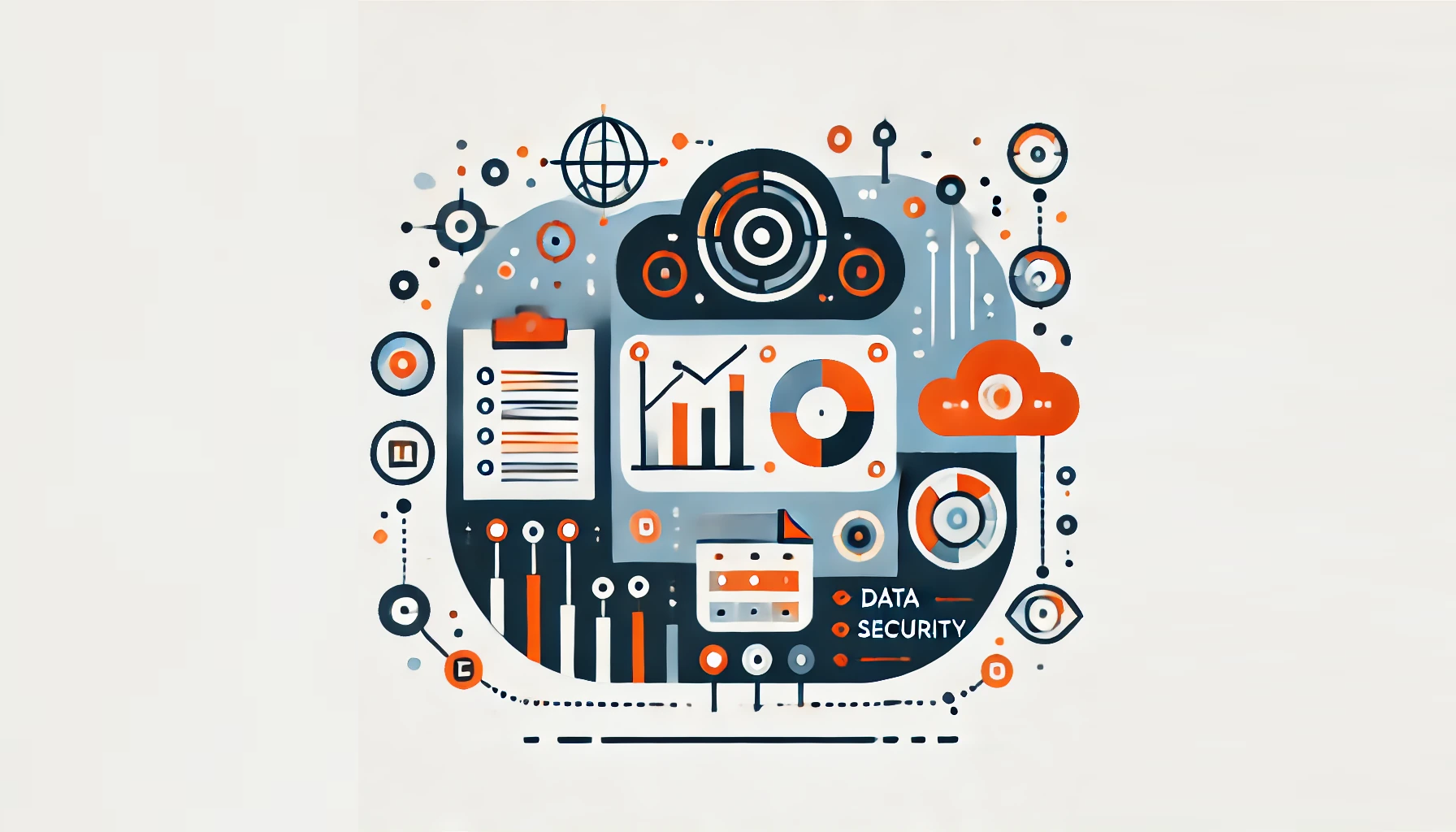
A recent Gartner poll of over 2,500 executive leaders revealed that 45% have increased their AI investments in response to the buzz surrounding ChatGPT. At DataSunrise, we’re keeping pace with this trend. You’ve probably read our previous article on the AI-based tools for synthetic (random or fake) data generation. This article concerns more on the topic of synthetic data generation with DataSunrise and some other free available tools.
Whether for testing, training, or development, obtaining real-world data poses challenges. Privacy concerns, data availability issues, and regulatory restrictions often hinder access to real data. This is where random data generation comes into play. It offers a solution by creating artificial data that mimics real data characteristics without compromising privacy or security.
What is Synthetic Data?
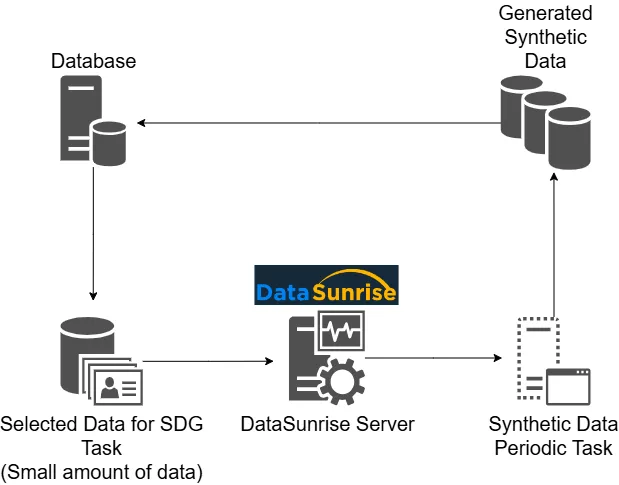
Synthetic data is artificially generated data that resembles real-world data in terms of statistical properties, patterns, and structures. It does not contain any actual information about individuals or entities. Instead, you create this data using algorithms and mathematical models to maintain authenticity while avoiding the risks associated with handling sensitive data.
Capabilities of DataSunrise in Synthetic Data Generation
DataSunrise offers a robust random data generation feature that accurately mimics real-life data. People use this feature for various business purposes, from developing and testing to improving machine learning algorithms. Let’s delve into the capabilities of DataSunrise in the field of synthetic data generation.
Data Privacy and Security Testing
One of the primary applications of data is in data privacy and security testing. Organizations, especially in sectors like finance, healthcare, and legal, can use synthetic data to assess their security systems without exposing real sensitive information. For example, a financial institution can generate synthetic transaction data to test its fraud detection systems.
Machine Learning Model Training
Industries increasingly use fake data to train machine learning models. This approach ensures that the privacy of actual data is not compromised. For instance, a healthcare company can generate synthetic patient records to train a predictive model for disease diagnosis without breaching patient confidentiality.
Software Development and Testing
Synthetic data is invaluable in software development. It provides realistic datasets for creating and evaluating applications, particularly in industries like telecommunications. For example, a telecom company can generate synthetic call records to test its billing software.
Healthcare Analytics
In healthcare, such data enables researchers and data scientists to conduct studies and experiments without breaching patient confidentiality. For instance, a research team can generate synthetic patient data to study the effects of a new drug.
How to Generate Synthetic Data with DataSunrise
DataSunrise simplifies the process of random data generation, making it easy to integrate data into various workflows. Here’s a step-by-step guide on how to generate data using DataSunrise.
Step 1: General Settings
Go to the Configuration – Periodic Tasks. Click +New task. In the General Settings subsection, set the name for your Periodic Task. Select the type of the task – Synthetic Data Generation – and on which server to start (optional).
Step2: Select Database Instance
In the Synthetic Data Generation subsection, select the database instance. PostgreSQL instance is selected on the figure below.
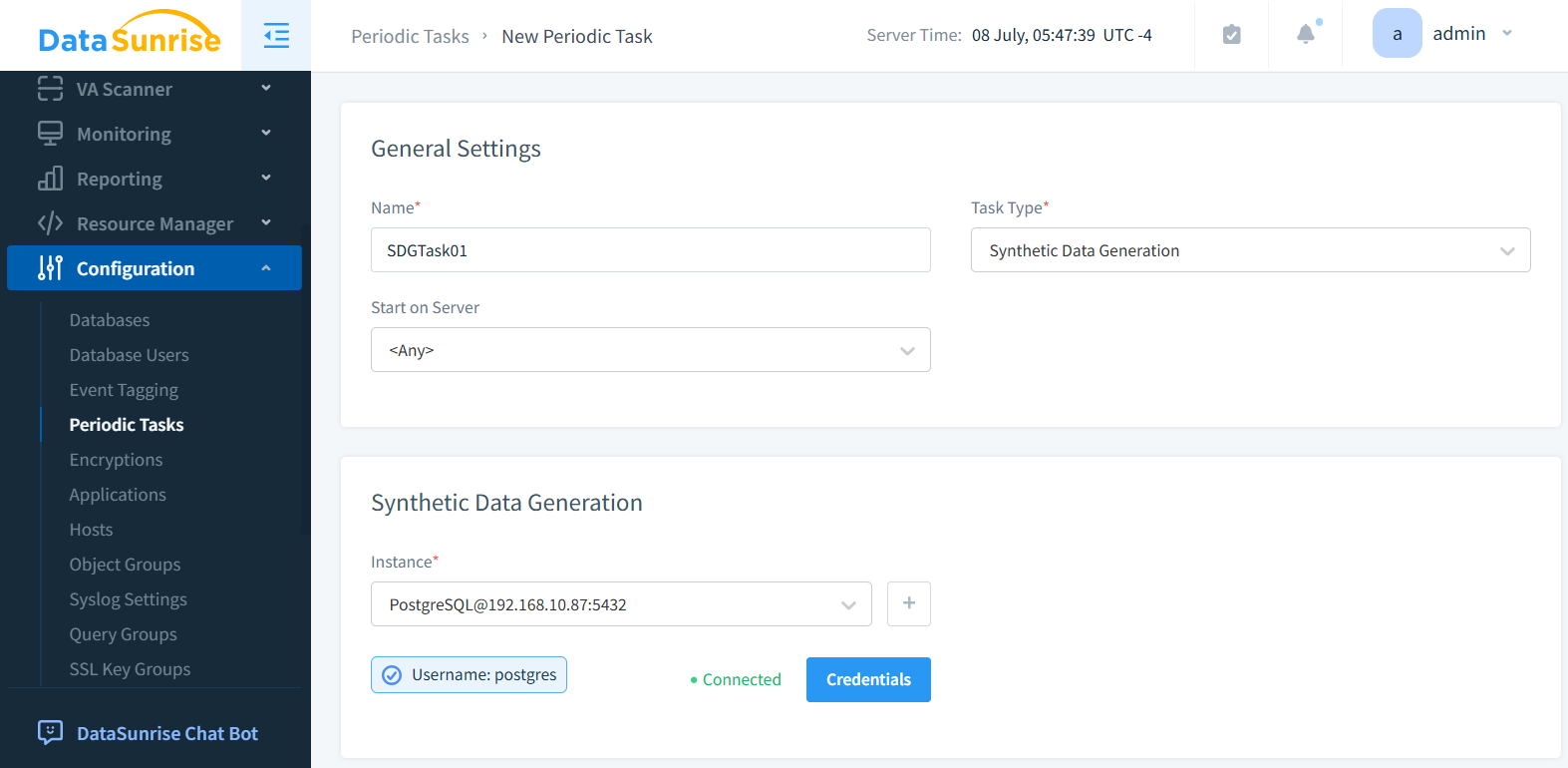
Step 3: Generated Tables
In the Generated Tables subsection, select the needed checkboxes (e.g., Empty Target Table and Skip Table Generation on Error). Click +Select to open a window where you can select the database objects you need. Choose a database, schema, table, and column for which synthetic data will be generated. After making your selections, click Save.
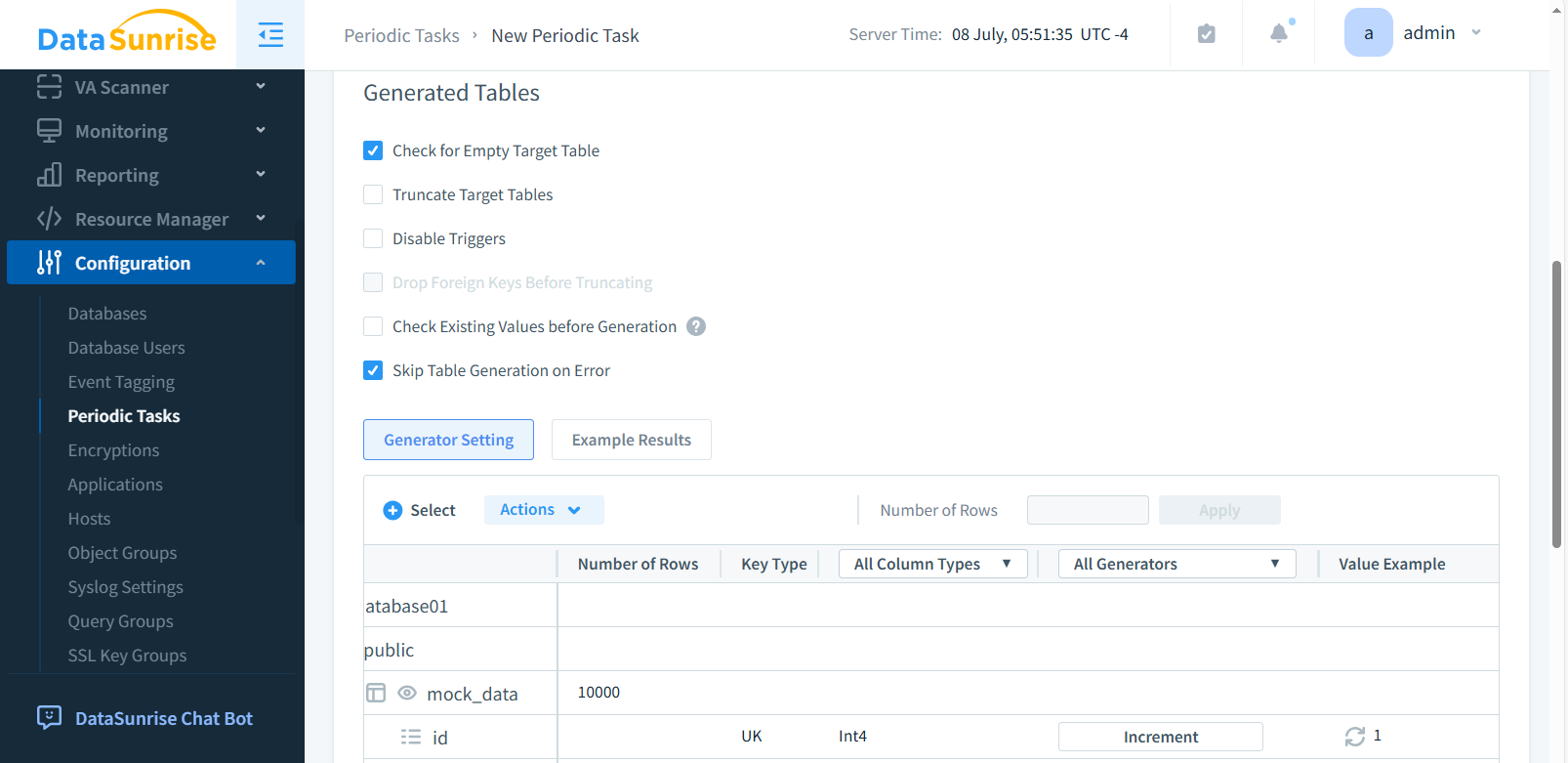
Step 4: Selecting Data Generators (optional)
In the All Generators column, you can select or create the generator. In the Example Results section, you will see the list of generated data. After everything is done, click Apply or Save. This is optional as the system assigns default generators to the columns selected.
If you want to create your own specific generator (before creating Synthetic Data Generation task), go to the Configuration – Generators, and click +Create Generator. Select a generator type and specify its parameters. Click Save, and you will be able to apply your generator in the Synthetic Data Generation Task.
‘Number of rows’ on top of the table becomes active when the column is selected.
Step 5: Saving and running the task
Here you can see the Periodic Tasks with Synthetic Data Generation Task along with some User behavior periodic task created earlier.
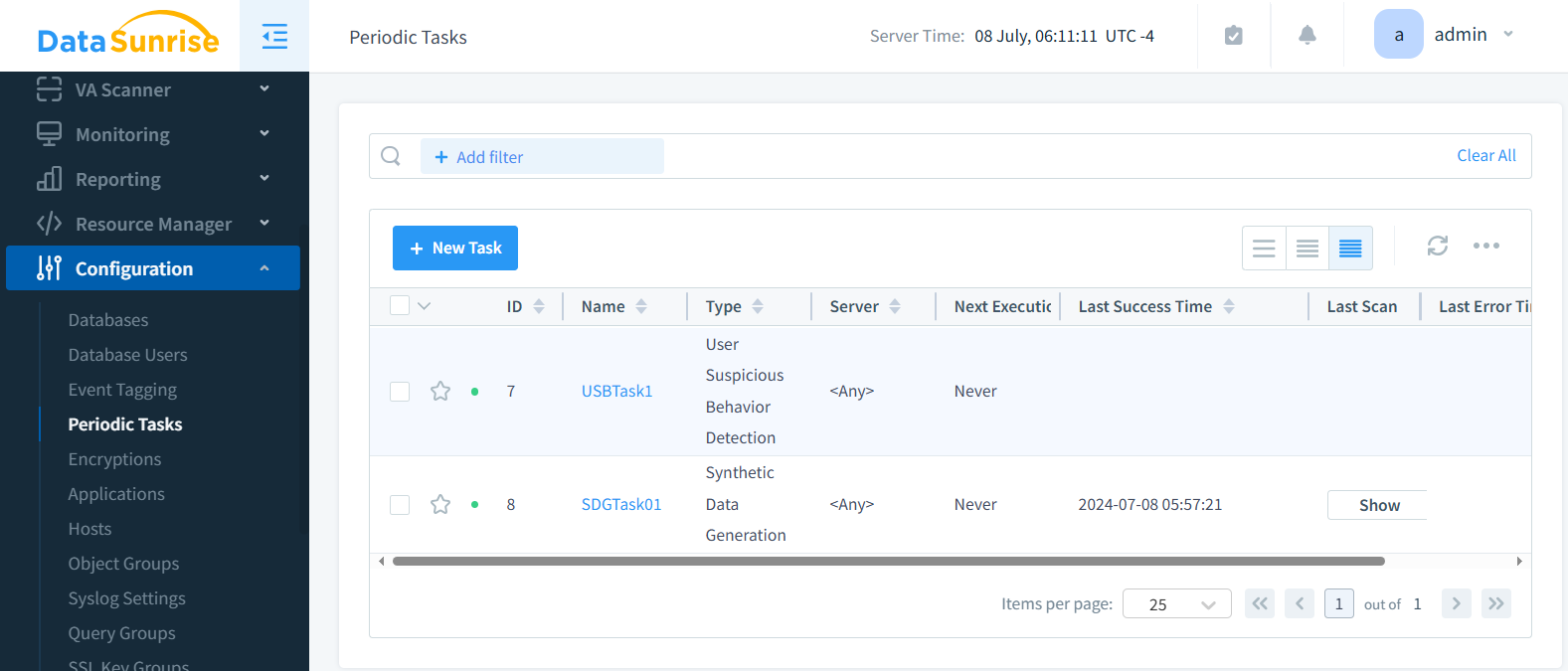
The task is ready now. Run the task as you need or make it run periodically.
Online Tools and Open-Source Solutions
DataSunrise offers highly flexible and robust control over random data generation, along with top-tier database security solutions that provide the largest coverage of databases and cloud warehouses available in the market. However, what about free options? Several online tools and open-source libraries are available for generating fake data at no cost. Let’s explore some popular options:
SDV (Synthetic Data Vault)
We briefly discussed this topic in our previous article on AI data generation. There, we mentioned that CTGAN is a component of SDV (Synthetic Data Vault). To recap, SDV is an open-source Python library for generating multi-table relational data. It uses machine learning to create artificial data that maintains the statistical properties of the original dataset. To install using pip use the following command:
pip install sdv
Example usage:
from sdv.datasets.demo import download_demo from sdv.single_table import GaussianCopulaSynthesizer # Download the demo dataset real_data, metadata = download_demo( modality='single_table', dataset_name='fake_hotel_guests' ) # Create and fit the synthesizer synthesizer = GaussianCopulaSynthesizer(metadata) synthesizer.fit(real_data) # Generate fake data synthetic_data = synthesizer.sample(num_rows=500) # Display the first few rows of the generated data print(synthetic_data.head())
This script uses the GaussianCopula synthesizer from SDV to generate synthetic data based on the statistical properties of a real dataset.
The result may look like this:
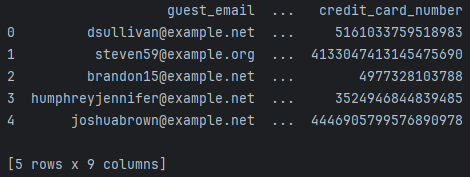
CTGAN (Conditional Tabular GAN)
CTGAN is a GAN-based model specifically designed for generating synthetic tabular data. It’s particularly useful for complex datasets with mixed data types.
Please see our previous article on AI-related tools for synthetic data generation for CTGAN code sample.
Mockaroo
Mockaroo is a Ruby-written web-based tool that allows you to generate realistic random data in various formats (CSV, JSON, SQL, etc.) without programming. It offers a user-friendly interface and supports custom data schemas. Free access is limited with 1000 rows of data.
Best Practices for Fake Data Generation
To ensure high-quality mock data:
- Understand your data requirements and use case
- Choose the appropriate generation method based on your needs
- Validate the generated data against your original dataset or requirements
- Ensure data privacy by avoiding the inclusion of sensitive information
- Continuously refine your generation process based on feedback and results
Conclusion
Synthetic data generation provides a valuable solution for organizations looking to work with realistic data while safeguarding privacy and security concerns. DataSunrise simplifies this process, making it easy to integrate artificial data into various workflows. However, it’s essential to validate the effectiveness and reliability of synthetic data. Organizations should ensure that the generated data accurately represents the real data distribution and maintains the necessary relationships and dependencies.
In summary, data generation offers numerous advantages, from enhancing data privacy and security to improving machine learning models and software testing. With the DataSunrise Synthetic Data Generation feature, organizations can confidently navigate the data landscape and harness the power of generated data for their business needs.
For more information, visit our website or request an online demo.