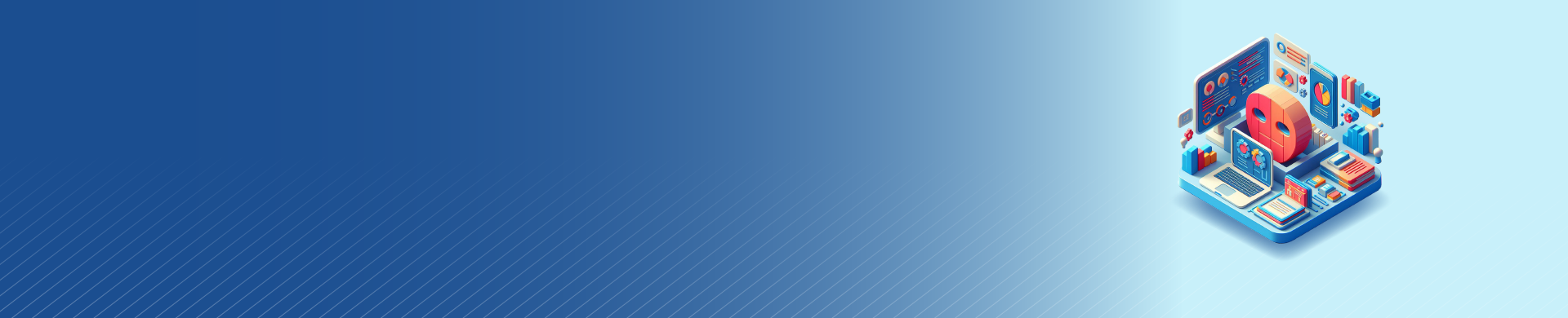
Types of Data Masking
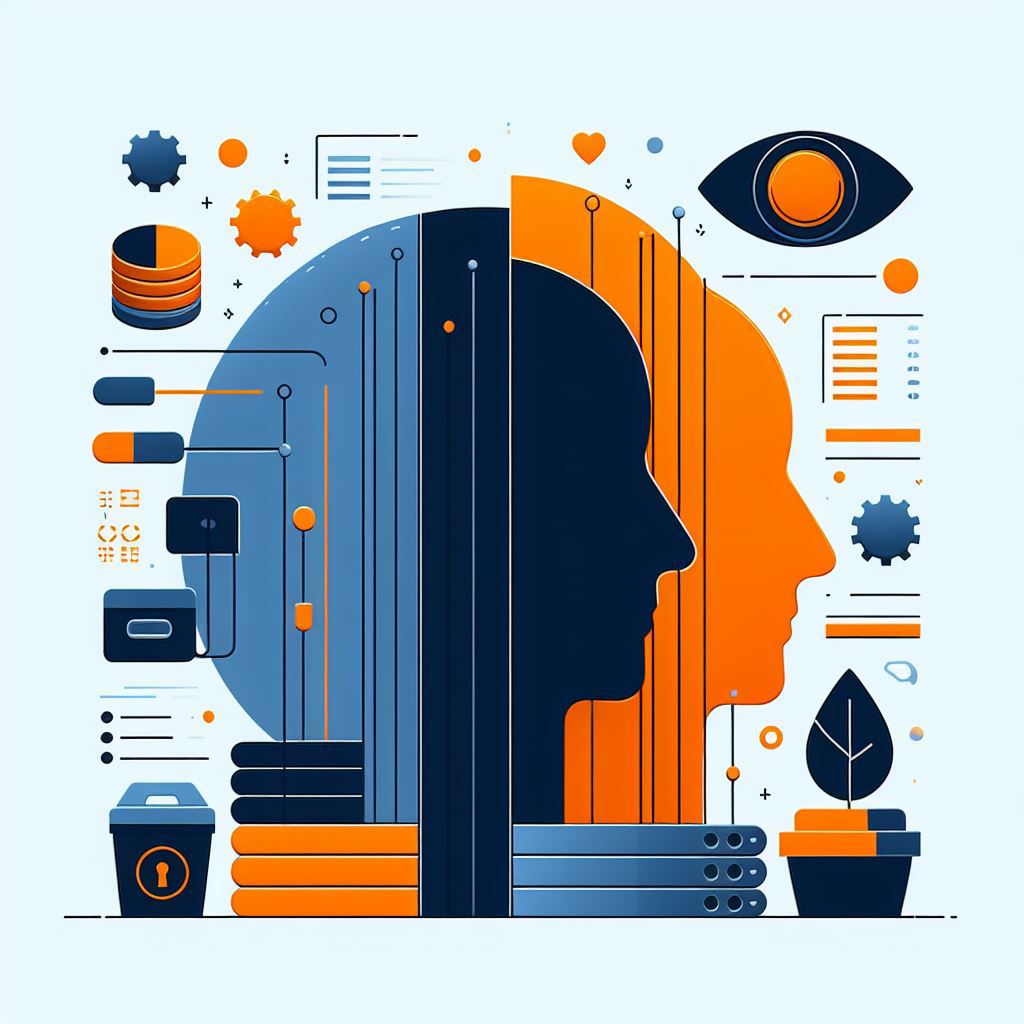
Protecting sensitive data is paramount for any organization. Data masking emerges as a crucial technique to ensure data privacy and security. This article delves into the various types of data masking, explaining their applications and differences. By understanding these methods, businesses can better protect their critical information from unauthorized access.
Data masking is when you make a fake version of a company’s data to keep important information safe. This technique is crucial for following privacy laws and protecting data in environments that need testing and analysis.
Types of Data Masking
Static Data Masking (SDM)
Static Data Masking involves creating a copy of the data and applying transformation techniques to mask sensitive information. This copy then replaces the original data in non-production environments. The data remains secure even if the environment is compromised because it is transformed before leaving the database.
Example of Static Data Masking: Imagine a healthcare database with patient records. Before using this data for software testing, a static data masking process replaces all patient names and IDs with fictitious but realistic entries. The data structure and format will stay the same, so applications can function as usual without revealing actual patient data.
Dynamic Data Masking (DDM)
The system applies Dynamic Data Masking in real-time as it processes data requests. Unlike SDM, it does not create a physical copy of the data. When querying the data, the system applies data masking rules to ensure that the original data in the database remains unchanged.
Example of Dynamic Data Masking: A financial analyst queries a database containing client financial records. DDM automatically hides account numbers and balances in query results. This ensures that analysts can only see the information they need for their analysis. It also prevents sensitive data from being exposed.
In-place Data Masking
Although not a stand-alone masking type, In-place masking is worth mentioning as an exception case for static masking. In-place masking occurs when the source production database and the target masked database are the same. This means that sensitive parts of your existing data are intentionally removed or masked. This procedure carries risks and should only be attempted if the database administrator is confident in the final result.
When Does Masking Take Place?
- Static Data Masking masks the data before moving it to a non-production environment.
- Dynamic Data Masking happens on-the-fly, during data retrieval.
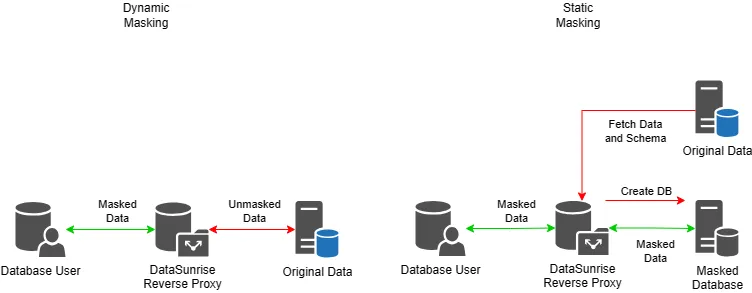
Nature of Data Changes in Masking
Data masking can be reversible or irreversible depending on the method used:
- Reversible Masking: This is often used when there is a need to revert to the original data, typically under secure conditions.
- Irreversible Masking: This method is used when there is no need to access the original data again, enhancing security.
Note that dedicated software like DataSunrise does not change the data at rest for dynamic data masking. This type of masking works in reverse proxy mode. Instead of the actual database, the queries to the database are processed by the DataSunrise proxy. The user-end software works with the database connection as usual.
In the case of static masking, DataSunrise copies data to a different database by default. This minimizes the risk of data loss.
Masking Methods
These are not masking types but you should take care of masking methods in some situations. Fake data must mimic the format not only to mislead the attacker. This is also a way to support old software which is sensitive to the data format.
Substitution
Substitution involves replacing the original data with fictitious but realistic values. You must generate fictitious data beforehand. This technique maintains the format and structure of the data while ensuring that the masked values are not reversible. Example:
Original Data: John Doe Masked Data: James Smith
Shuffling
Shuffling rearranges the values within a column, breaking the relationship between the masked data and the original data. This technique is useful when you need to maintain the distribution and uniqueness of the data. Example:
Original Data: John Doe, Jane Smith, Alice Johnson Masked Data: Alice Johnson, John Doe, Jane Smith
Encryption
Encryption involves converting the original data into an unreadable format using a cryptographic algorithm and a secret key. You can only decrypt the masked data with the corresponding key, which makes it reversible. People commonly use encryption when they need to recover the original data. Example:
Original Data: John Doe Masked Data: Xk9fTm1pR2w=
Tokenization
Tokenization replaces sensitive data with a unique, randomly generated token. The system securely stores the original data in a token vault. The token serves as a reference to retrieve the data when needed.
Companies commonly use tokenization to protect credit card numbers and other sensitive financial data. Example:
Original Data: 1234-5678-9012-3456 Masked Data: TOKEN1234
In the picture below you can see the masking methods selection in DataSunrise. This appears when you create a dynamic Masking rule using DataSunrise Web-based UI. The masking methods available may range through trivial ‘Empty String’ to more advanced format preserved ‘FF3 Encryptions’.
Masking with Native DBMS Tools: Pros and Cons
Database management systems (DBMS) often provide native tools, such as views and stored procedures. You can use them to implement data masking. While these tools offer some advantages, they also have limitations compared to dedicated data masking solutions. Let’s explore the pros and cons of using native DBMS tools for creating masking.
Pros
Familiarity: Database administrators (DBAs) and developers are often well-versed in using native DBMS tools. This familiarity can make it easier for them to implement masking using these tools without requiring additional training.
Integration: The database system Natively integrates DBMS tools. This allows for seamless interaction with the data. This integration can simplify the implementation process and ensure compatibility with existing database operations.
Performance: You can execute views and stored procedures directly within the database engine. This provides better performance compared to external masking solutions. This is especially beneficial when dealing with large datasets or complex masking rules.
Cons
Limited Functionality: Native DBMS tools may not offer the same level of functionality as dedicated data masking solutions. They may lack advanced masking techniques, such as format-preserving encryption or conditional masking. This can limit the effectiveness of the masking process.
Maintenance Overhead: Implementing masking using views and stored procedures requires custom development and ongoing maintenance. As the database schema evolves, we need to update the views and stored procedures accordingly. This can be time-consuming and prone to errors, especially in complex database environments.
Scalability Challenges: When using native DBMS tools for masking, the masking logic is tightly coupled with the database schema. Scaling the masking solution across multiple databases or adapting to changes in the data structure can be difficult. Dedicated masking solutions often provide more flexibility and scalability in handling diverse data sources and evolving requirements.
Security Concerns: Views and stored procedures are part of the database system. Users with appropriate privileges can access them. If not properly secured, there is a risk of unauthorized access to the masking logic or the unmasked data. Dedicated masking solutions often provide additional security measures and access controls to mitigate these risks.
Consistency and Standardization: When relying on native DBMS tools, the masking implementation may vary across different databases and teams. This lack of consistency can lead to disparities in the masked data and make it challenging to maintain a standardized masking approach across the organization. Dedicated masking solutions offer a centralized and consistent approach to masking, ensuring uniformity and compliance with data protection policies.
Creating Masking Rules in DataSunrise
To implement data masking with DataSunrise, you can use either Web-based GUI or the Command Line Interface (CLI).
Example using CLI for dynamic masking rule (single line):
executecommand.bat addMaskRule -name script-rules -instance aurora -login aurorauser -password aurorauser -dbType aurora -maskType fixedStr -fixedVal XXXXXXXX -action mask -maskColumns 'test.table1.column2;test.table1.column1;'
This command creates a masking rule named “script-rules” that substitutes values in the “test.table1.column2” and “test.table1.column1” columns of the “table1” table. You can see the DataSunrise CLI Guide for details.
Conclusion and Summary
Data masking is a vital security measure that helps organizations protect sensitive information. Understanding the different types of data masking and when to use them can significantly enhance your data security strategy. Static and Dynamic Data Masking each have their roles depending on the sensitivity of the data.
While native DBMS tools like views and stored procedures can be used for creating masking. However they have limitations compared to dedicated data masking solutions. Organizations should carefully evaluate their masking requirements, considering factors such as functionality, scalability, security, and maintainability, before deciding on the appropriate approach.
Dedicated masking solutions, like DataSunrise, offer comprehensive features, flexibility, and ease of use. This makes them a preferred choice for organizations looking to implement robust and reliable data masking practices. DataSunrise provides a wide range of masking techniques, supports multiple databases. It also offers a centralized Web-based management console for defining and applying masking rules consistently across the enterprise.
Come join our team for an online demonstration to see how our solutions can effectively protect your data.
Note on DataSunrise: DataSunrise’s exceptional and flexible tools not only provide robust security but also ensure compliance and efficient data management. Join us for an online demo to explore how we can assist in safeguarding your data assets.