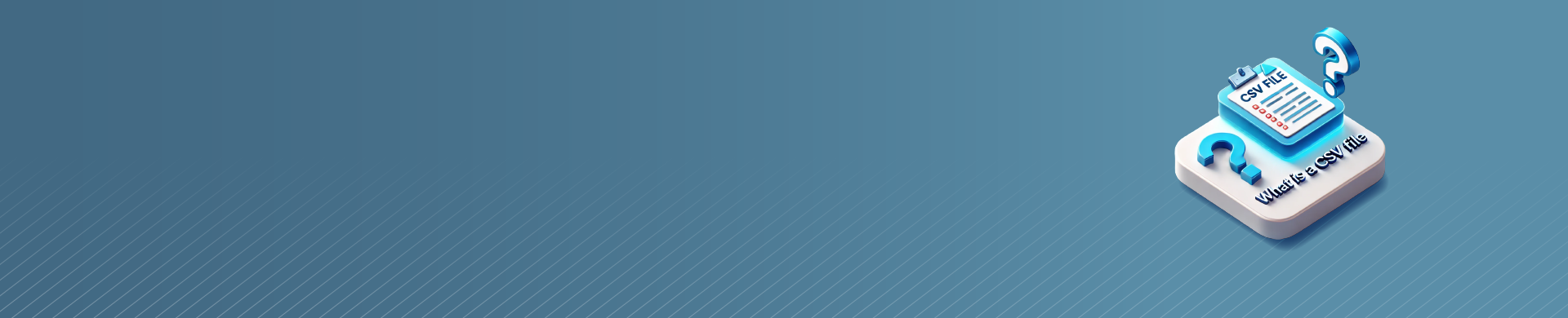
What is a CSV File?
Introduction: The Humble CSV File
Did you know that CSV files have been around since the early days of computing? In the 1970s and early 1980s, IBM’s Fortran 77 language introduced the character data type, which enabled support for comma-separated input and output. These simple yet powerful files have stood the test of time, remaining a popular choice for data exchange even in our modern, tech-driven world. Let’s dive into the world of comma-separated files and explore why they continue to be a go-to format for many data professionals and casual users alike.
We previously described DataSunrise’s capabilities for handling semistructured data in JSON files. Check out that information to learn more about DataSunrise’s data security features.
With DataSunrise you can mask and discover the sensitive data in CSV files stored locally or in S3 storage. Here is the masking example.
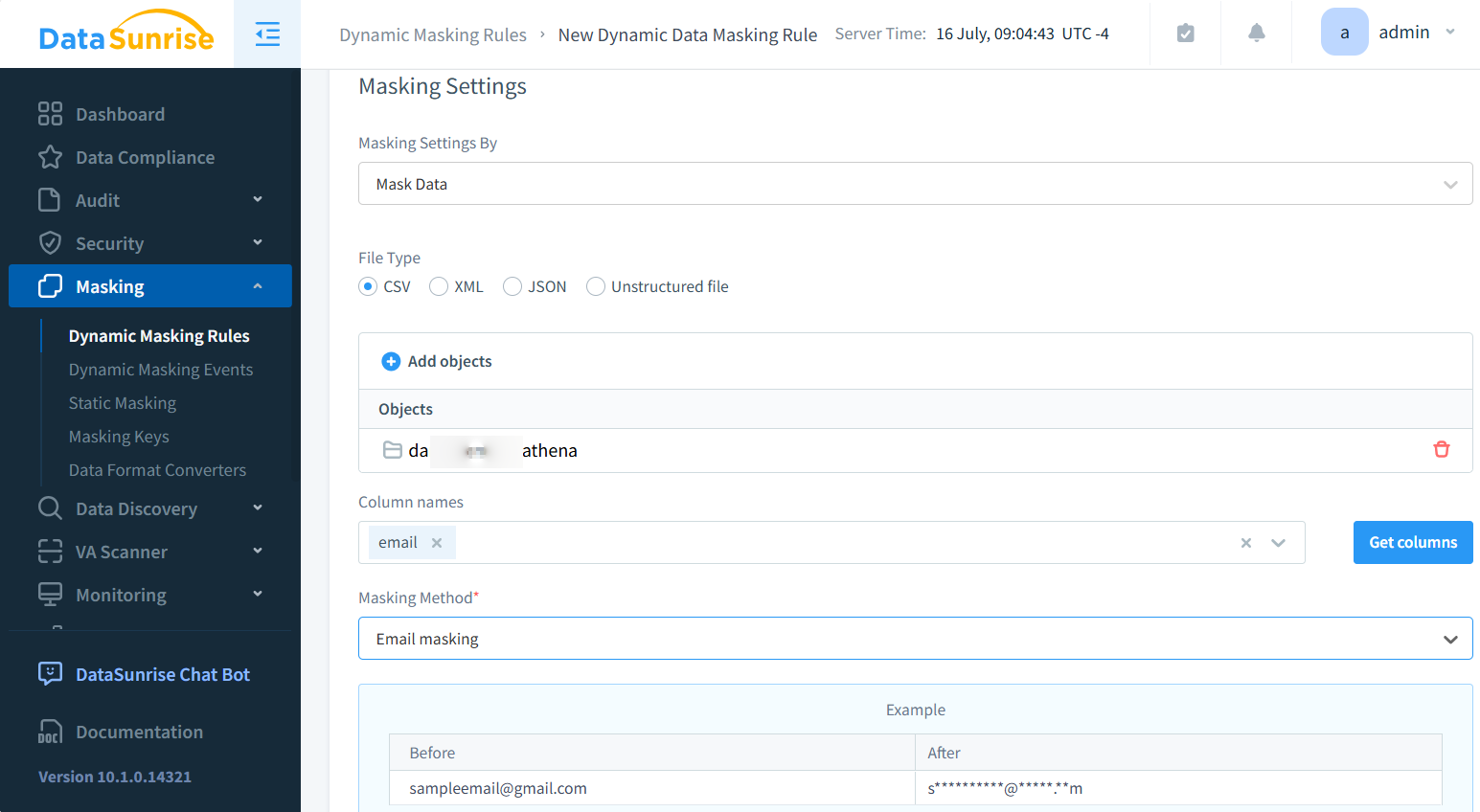
After a simple setup, you can access (download) the masked CSV files through DataSunrise’s S3 proxy using specialized software like S3Browser. Proper configuration of the proxy settings is required in the client software. The result is as follows:

In the vast ecosystem of file formats, the CSV file continues to stand out for its clarity and versatility. A CSV (Comma-Separated Values) file is a plain text document designed to store tabular data. Each line represents a row, and commas divide the values within. This simple structure makes the CSV file format incredibly easy to read, generate, and process across operating systems and applications.
What is a CSV File?
A CSV file (Comma-Separated Values file) is a plain text document that stores tabular data in a structured format. Each line in the file represents a row of data, and values within each row are separated by commas. This simple format makes CSV files ideal for exchanging data between different applications and platforms.
The file extension for this format is typically “.csv” – for example, “data.csv” or “report.csv”. When opened in a text editor, the content appears as rows of text with commas dividing each value. However, when imported into spreadsheet software like Microsoft Excel or Google Sheets, the data automatically organizes into rows and columns.
CSV files can contain various types of data, including text, numbers, and dates. While commas are the traditional separators (hence the name), other characters like semicolons, tabs, or pipes can also be used as delimiters in some implementations. The first row often contains column headers that describe the data in each column, though this is not required by the format.
Unlike advanced spreadsheet formats, a CSV file does not support embedded objects, multiple tabs, or formatting features. Its minimalist structure is both a limitation and an advantage—ideal for lightweight CSV data exchange, but not meant for complex visual reports or analytical models.
Why Use CSV Files?
CSV files offer several advantages that contribute to their widespread use:
- Simplicity: The format is easy to understand and work with, even for non-technical users. You can open it in Notepad or Notepad++ (any text editor).
- Compatibility: Files can be opened and edited by a wide range of software, from spreadsheet applications to text editors.
- Data exchange: They serve as a universal format for transferring data between different systems and applications.
- Size efficiency: Files are typically smaller than their binary counterparts, making them ideal for storing and transmitting large datasets.
Here is a comparison table of data formats used in Big Data and Machine Learning, highlighting the role of comma-separated files in data processing.
Format | Big Data | Machine Learning | Pros | Cons |
---|---|---|---|---|
CSV | Common for data exchange, less common for storage | Often used for small to medium datasets | Simple, human-readable, widely supported | Not efficient for large datasets, no schema enforcement |
Parquet | Very common for storage and processing | Good for large datasets and feature stores | Columnar storage, efficient compression | Not human-readable, requires special tools to view |
Avro | Common for data serialization | Less common, but used in some pipelines | Schema evolution, compact binary format | More complex than CSV, not as efficient as Parquet for analytics |
JSON | Common for APIs and document stores | Used for storing metadata and small datasets | Flexible, human-readable, widely supported | Less efficient storage than binary formats |
TFRecord | Not commonly used | Specific to TensorFlow, common in ML pipelines | Efficient for large datasets, good with TensorFlow | Not widely supported outside TensorFlow ecosystem |
CSV Example
Let’s look at a simple CSV example to illustrate its structure:
Name, Age, City John Doe, 30, New York Jane Smith, 25, London Bob Johnson, 35, Paris
This example shows how data is organized in a CSV file, with each line representing a record and commas separating the values.
Working with CSV Files in Python
Python offers built-in modules and libraries for processing CSV files, making it one of the most popular languages for working with tabular data in CSV format.
Python’s csv module offers straightforward methods for reading and writing CSV files. Here’s a basic example:
import csv # Reading a file with open('data.csv', 'r') as file: csv_reader = csv.reader(file) for row in csv_reader: print(row) # Writing to a file with open('output.csv', 'w', newline='') as file: csv_writer = csv.writer(file) csv_writer.writerow(['Name', 'Age', 'City']) csv_writer.writerow(['Alice', '28', 'Berlin'])
This code demonstrates how to read from and write to CSV files using Python’s built-in csv module.
Using Pandas
For more advanced data manipulation, the pandas library is an excellent choice. It provides powerful tools for working with semi-structured data, including CSV files:
import pandas as pd # Reading a file df = pd.read_csv('data.csv') # Displaying the first few rows print(df.head()) # Writing to a file df.to_csv('output.csv', index=False)
Pandas makes it easy to perform complex operations on CSV data, such as filtering, sorting, and aggregating. You can easily save the data back in CSV later.
The Pros and Cons of Comma-separated Files
While CSV files are widely used, it’s important to understand their strengths and limitations:
Advantages
- Human-readable: Comma-separated files can be easily viewed and edited in text editors.
- Lightweight: They have a small file size compared to many other formats.
- Widely supported: Most data processing tools and programming languages can work with CSV files.
Disadvantages
- Limited data types: Text files don’t inherently support complex data types or structures.
- No standardization: There’s no official standard for CSV files, leading to potential compatibility issues. There are no required columns or mandatory delimiters.
- Data integrity: Comma-separated files don’t have built-in error checking or data validation mechanisms. Big Data formats (like Parquet) include built-in checksums for data blocks.
Binary Formats: When and Why They’re Better
While CSV files excel in many scenarios, binary formats can be advantageous in certain situations:
- Performance: Binary formats are often faster to read and write, especially for large datasets.
- Data types: They can preserve complex data types and structures more accurately.
- Compression: Binary formats typically offer better compression ratios, saving storage space.
- Security: Some binary formats provide options for encryption and access control.
Examples of binary formats include HDF5, Parquet, and Avro. These formats are particularly useful in big data environments where performance and data integrity are crucial.
CSV Files in Data Exchange
CSV files play a vital role in data exchange across various industries and applications:
- Business intelligence: Companies often use text files to transfer data between different BI tools and databases.
- Scientific research: Researchers frequently share datasets in this format for easy analysis and collaboration.
- Web applications: Many web services allow users to export data in comma-separated format for offline analysis or backup purposes.
- IoT and sensor data: Comma-separated text files are commonly used to log and transmit data from IoT devices and sensors.
The simplicity and universal nature of text files make them an ideal choice for these data exchange scenarios.
CSV Files in Enterprise Settings
CSV files remain crucial in enterprise data workflows. Many legacy systems rely on CSV for data imports. Financial institutions use CSV for daily transaction reports. Healthcare systems exchange patient data through secure CSV transfers. Data migration projects often begin with CSV exports. ETL pipelines frequently consume CSV as source data. Cloud storage vendors optimize for CSV storage and retrieval. Regulatory compliance often requires CSV archives of critical data. Auditors commonly request data in CSV format for verification. CSV files serve as universal translators between incompatible systems. Their simplicity makes them ideal for scheduled automated data exchanges.
CSV Files in the Big Data Field
Comma-Separated Values files have a somewhat complex relationship with Big Data. Let me break this down for you:
- Popularity in certain contexts:
- Comma-separated file format is still widely used for data exchange and as an intermediate format in Big Data ecosystems.
- It’s often used for importing data into Big Data systems or exporting results for further analysis.
- Limitations for Big Data:
- CSV files don’t compress well, which can be an issue when dealing with very large datasets.
- They lack built-in schema definitions, which can lead to data inconsistencies in large-scale operations.
- Parsing large text files can be slower compared to some binary formats.
- Preferred alternatives:
- For Big Data operations, formats like Parquet, Avro, or ORC are often preferred.
- These formats offer better compression, schema evolution, and faster processing speeds.
- Use cases where comma-separated fies are still relevant:
- Data ingestion: Many systems still accept comma-separated values as an input format.
- Legacy systems: Some older systems may still rely on these files for data exchange.
- Simple datasets: For smaller or less complex datasets within a Big Data ecosystem, CSV might still be used.
- Hybrid approaches:
- Some Big Data workflows might use CSV for initial data ingestion or final output, while using more optimized formats for intermediate processing steps.
When to Use a CSV File vs Binary Format
Use Case | Best Format | Why |
---|---|---|
Data Exchange Between Systems | CSV | Simple, universally supported, human-readable |
Large-scale analytics or machine learning | Parquet / Avro | Compression, schema support, efficient parsing |
Small-scale reports or logs | CSV | Easy to export, import, and read without special tools |
Conclusion: The Enduring Value of CSV Files
CSV files continue to be a valuable tool in the data professional’s toolkit. Their simplicity, versatility, and widespread support make them an excellent choice for many data exchange and storage scenarios. While binary formats offer advantages in certain situations, the humble text file remains a go-to solution for quick and easy data sharing across platforms and applications.
As we’ve explored, working with comma-separated files in Python is straightforward, whether you’re using core Python or more advanced libraries like pandas. This accessibility contributes to the ongoing popularity of CSV files in data analysis and processing tasks.
For those dealing with sensitive data in CSV files or other semi-structured formats, DataSunrise offers user-friendly and flexible tools for database security. Our solutions include NLP-based data discovery, which can be particularly useful when working with comma-separated files containing potentially sensitive information. To learn more about how DataSunrise can enhance your data security measures, visit our website for an online demo and explore our comprehensive database security solutions.