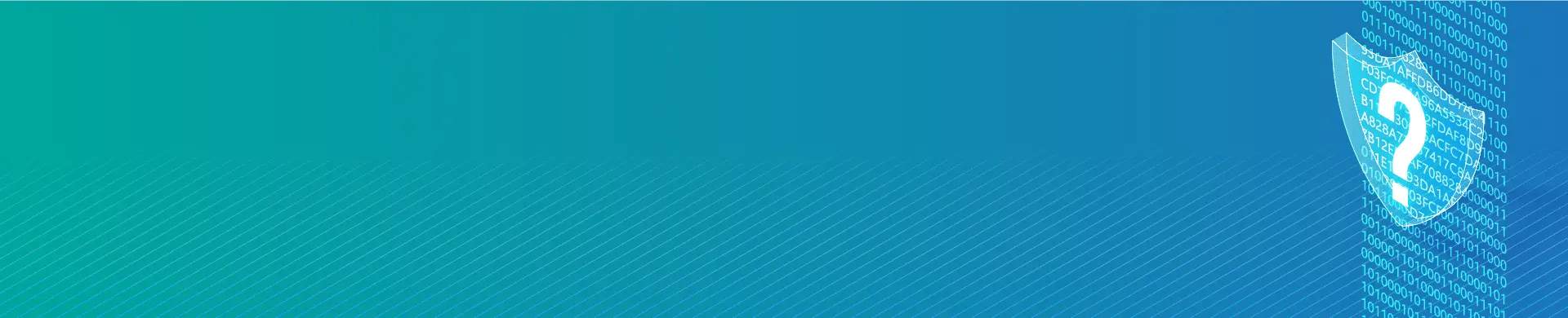
What is Data Masking?
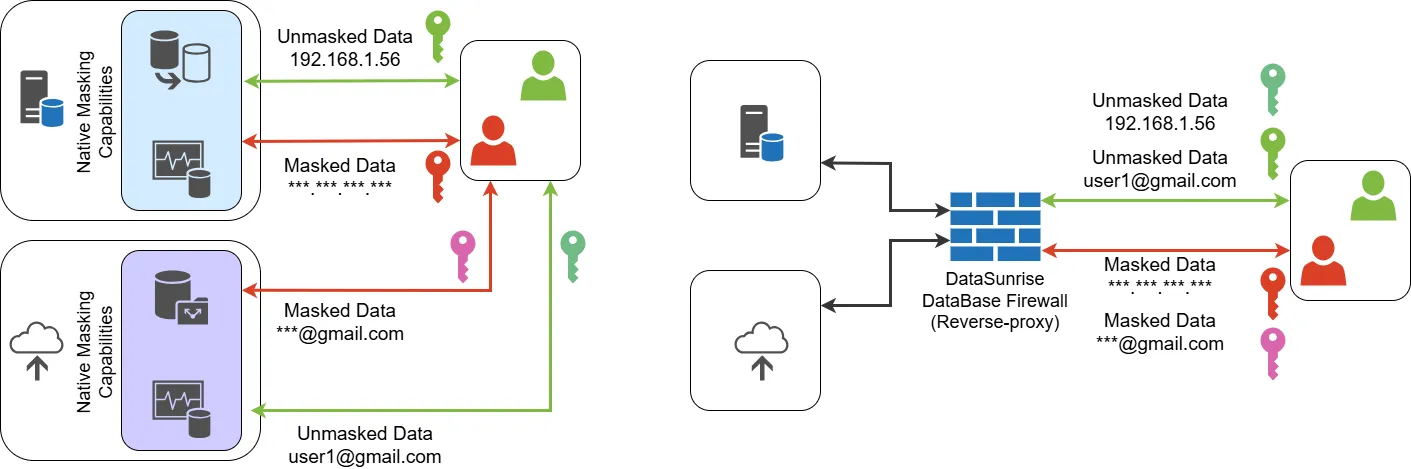
Data masking, also known as data obfuscation, is the process of replacing sensitive information with realistic but inauthentic data. Its primary purpose is to protect confidential information, such as personal data, stored in proprietary databases. However, effective masking strikes a balance between security and utility, ensuring that the obfuscated data remains suitable for essential corporate activities like software testing and application development.
Masking proves invaluable in scenarios such as:
- a company needs to give access to its database(s) to outsource and third-party IT companies. When you are masking data, it’s very important to make it look and appear consistent so that hackers and other malicious actors think that they’re dealing with genuine data.
- a company needs to mitigate operators’ errors. Companies usually trust their employees to make good and secure decisions, however many breaches are a result of operators’ errors. If data is masked, the results of such errors are not so catastrophic. Also, it’s worth mentioning that not all operations in databases need the use of entirely real, accurate data.
- a company runs data-driven testing.
In this article we are going to look more closely at static masking, dynamic masking and in-place masking.
Examples of Masked Data
In the example below you can see how the Card column looked before masking:
SQL> select * from scott.emp; EMPNO ENAME JOB MGR HIREDATE CARD --------- --------- ---------- ------- --------- ------------------- 1 SMITH CLERK 0 17-DEC-80 4024-0071-8423-6700 2 SCOTT SALESMAN 0 20-FEB-01 4485-4392-7160-9980 3 JONES ANALYST 0 08-JUN-95 6011-0551-9875-8094 4 ADAMS MANAGER 1 23-MAY-87 5340-8760-4225-7182 4 rows selected.
And after masking:
SQL> select * from scott.emp; EMPNO ENAME JOB MGR HIREDATE CARD --------- --------- ---------- ------- --------- ------------------- 1 SMITH CLERK 0 17-DEC-80 XXXX-XXXX-XXXX-6700 2 SCOTT SALESMAN 0 20-FEB-01 XXXX-XXXX-XXXX-9980 3 JONES ANALYST 0 08-JUN-95 XXXX-XXXX-XXXX-8094 4 ADAMS MANAGER 1 23-MAY-87 XXXX-XXXX-XXXX-7182 4 rows selected.
DataSunrise lets you apply different masking methods to each field. You can choose from preset options or create custom masking rules for specific data types. Format-preserving masking maintains data structure while protecting sensitive information. This ensures masked data remains usable and retains its statistical properties.
Masking Method | Original Data | Masked Data |
---|---|---|
Credit card masking | 4111 1111 1111 1111 | 4111 **** **** 1111 |
Email masking | [email protected] | j***e@e*****e.com |
URL masking | https://www.example.com/user/profile | https://www.******.com/****/****** |
Phone numbers masking | +1 (555) 123-4567 | +1 (***) ***-4567 |
Random IPv4 address masking | 192.168.1.1 | 203.45.169.78 |
Random Date/Datetime with constant year for string column types | 2023-05-15 | 2023-11-28 |
Random Date/Datetime and Time from interval for string column type | 2023-05-15 14:30:00 | 2024-02-19 09:45:32 |
Masking by empty, NULL, substring value | Sensitive Information | NULL |
Masking by fixed and random values | John Doe | Anonymous User 7392 |
Masking using a custom function | Secret123! | S****t1**! |
Mask first and last chars of strings | Password | *asswor* |
Masking any sensitive data in a plain text | My SSN is 123-45-6789 and my DOB is 01/15/1980 | My SSN is XXX-XX-XXXX and my DOB is XX/XX/XXXX |
Masking by values from predefined dictionaries | John Smith, Software Engineer, New York | Ahmet Yılmaz, Data Analyst, Chicago |
Data Masking Steps
When it comes to practical implementation, you need the best strategy that works within your organization. Below are the steps you need to take to make masking effective:
- Find your sensitive data. The first step is to recover and identify data that may be sensitive and require protection. It’s better to use a special automated software tool for that, like DataSunrise sensitive data discovery with using of table relations.
- Analyze the situation. At this stage the data security team should understand where the sensitive data is, who needs access to it and who doesn’t. You can use role-based access. Everyone who has a certain role can see an original or masked sensitive data.
- Apply masking. One should bear in mind that in very large organizations, it isn’t feasible to assume that just a single masking tool can be used across the entire company. Instead, you might need different masking types.
- Test masking results. This is the final step in the process. Quality assurance and testing are required to ensure that the masking configurations give the required results.
Data Masking Types
For more detailed information on the masking types and their implementations using both native and third-party solutions, please visit our YouTube channel and explore our comprehensive masking playlist.
Dynamic Masking
Dynamic Masking is a process of masking data at the moment a query to a database with real private data is made. It is done through modifying the query or the response. At this data is masked on the fly, that is, without saving it to a transitional data storage.
Static Masking
As the name suggests, when masking data statically database administrators need to create a copy of the original data and keep it somewhere safe and replace it with a fake set of data. This process involves duplicating the content of a database into a test environment, which the organization can then share with third-party contractors and other external parties. As a result, original sensitive data needing protection stays in the production database and a masked copy is moved into the test environment. However perfect it may seem to work with third-party contractors using static masking, for applications needing real data from production databases statically masked data may be a big problem.
In-Place Masking
In-place masking like static masking also creates test data based on real production data. This process usually consists of 3 main steps:
- Copying production data as is to a test database.
- Removing redundant test data to decrease data storage volume and speed up testing processes.
- Replacing all PII data in a test database with masked values – this step is called in-place masking.
The way of copying of production data is left out of scope of in-place data masking itself. For example, it can be an ETL procedure or backup-recovery of a production database or something else. The most important thing here is that in-place masking is applied to a copy of a production database to mask the PII data it contains.
Conditions Data Masking Should Meet
As it was mentioned earlier any data involved in masking has to remain meaningful at several levels:
- The data has to remain meaningful and valid for the application logic.
- The data must undergo enough changes so that it can’t be reverse-engineered.
- The obfuscated data should remain consistent across multiple databases within an organization when each database contains the specific data element being masked.
Data Masking with DataSunrise
Masking is a crucial feature of any data security solution. We’re proud to offer DataSunrise masking capabilities, which provide one of the easiest-to-use yet most robust and full-fledged masking solutions on the market. In the picture below, you can see the masking setup for an email field. There are dozens of masking types available. You simply select the database and the data to mask (or the unstructured data location), set the type of masking, and your data is ready to pass regulatory compliance checks.
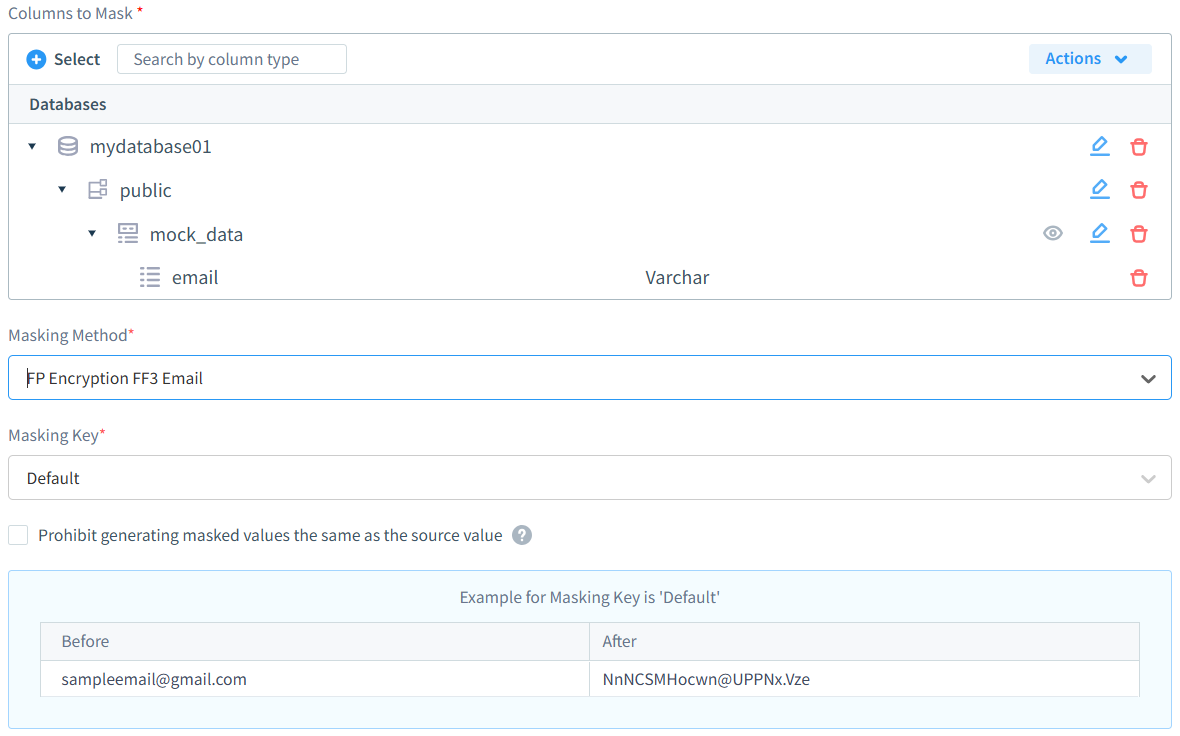
Conclusion
DataSunrise provides you with a possibility of static and dynamic data masking to protect your data (also masking XML, JSON, CSV, and unstructured text on Amazon S3). Moreover, data discovery with table relations will be an indispensable additional tool in the protection of your data. Our security suite guarantees the protection of data in your databases in the Cloud and on-Premises. Try now all our capabilities to be sure that everything is under your control.